A White Paper on How Quantum Machine Learning Is Ready For Primetime in Healthcare
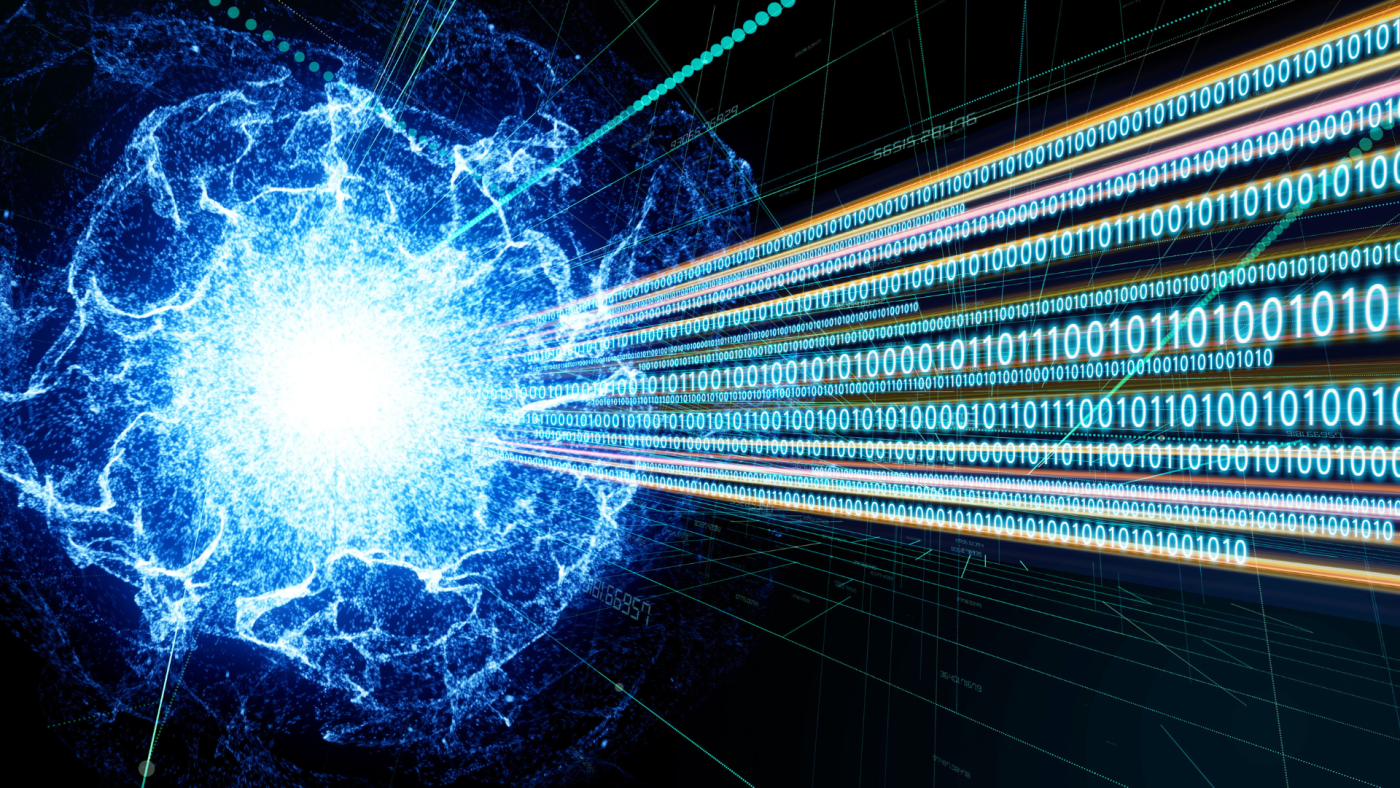
Authors: Amina H Khalpey, PhD, Antoni Macko, PhD, Zain Khalpey, MD, PhD, FACS
Quantum computing has long been touted as the next revolution in computing technology, offering unprecedented processing power and the ability to solve problems beyond the reach of classical computers. Machine learning has also been making significant strides in healthcare, with applications ranging from drug discovery to medical imaging analysis. Combining these two technologies, Quantum Machine Learning (QML) has the potential to transform healthcare by providing new insights into complex biological processes and enabling more accurate predictions of patient outcomes.
We argue that it is prime time to make this happen, as the convergence of these two technologies could lead to a new era of precision medicine. In this blog post, we explore the current state of QML in healthcare, highlighting key developments and challenges, and discuss the potential impact of QML in the next five years.
Introduction:
Healthcare is a critical sector that has a direct impact on the quality of life of individuals and the economies of nations. The healthcare industry faces numerous challenges, including the needs to: improve diagnosis and treatment of diseases, reduce healthcare costs, and improve patient outcomes. Machine learning has been shown to be a powerful tool in healthcare, helping to identify patterns in large datasets and making predictions about patient outcomes. However, machine learning algorithms are limited by the processing power of classical computers. Quantum computing, with its ability to perform calculations exponentially faster than classical computers, has the potential to overcome these limitations and transform the healthcare industry.
Current State of QML in Healthcare:
Quantum computing is still in its infancy, with few practical applications outside of research labs. However, in recent years, significant progress has been made in developing quantum algorithms for machine learning applications. These algorithms take advantage of the unique properties of quantum computing, such as superposition and entanglement, to perform calculations much faster than classical algorithms. One of the most promising applications of QML in healthcare is in drug discovery.
Drug discovery is a complex and time-consuming process that involves screening large databases of potential drug candidates to identify those that are most likely to be effective. QML has the potential to accelerate this process by identifying new drug targets and predicting the efficacy of potential drugs. In a recent study, researchers used a quantum algorithm to screen a database of six million drug-like molecules and identified several new drug candidates for treating Alzheimer’s disease. This study demonstrates the potential of QML to revolutionize drug discovery and improve the lives of millions of patients.
Another promising application of QML in healthcare is in medical imaging analysis. Medical imaging modalities such as MRI and CT scans produce large datasets that are difficult to analyze using classical algorithms. QML algorithms can rapidly analyze these datasets and identify patterns that are invisible to classical algorithms. In a recent study, researchers used a quantum algorithm to analyze MRI data from patients with brain tumors and identified a new biomarker that could be used to predict patient outcomes. This study demonstrates the potential of QML to improve patient outcomes by enabling more accurate predictions of disease progression.
Challenges of QML in Healthcare:
Despite the potential of QML in healthcare, there are several challenges that must be overcome before it can become a practical tool for clinicians. One of the biggest challenges is the limited availability of quantum hardware. Quantum computers are still in the early stages of development, and there are currently few commercial quantum computers available for researchers to use. This limits the ability of researchers to develop and test QML algorithms.
Another challenge is the difficulty of programming quantum computers. Quantum computing is fundamentally different from classical computing, and requires a new approach to programming. Many researchers are still learning the basics of quantum computing, and there is a shortage of skilled quantum programmers.
Finally, there is the challenge of integrating QML algorithms into clinical workflows. Healthcare is a highly regulated industry, and any new technology must meet rigorous safety and efficacy standards before it can be used in clinical practice. QML algorithms must be tested in clinical trials and validated against existing clinical standards before they can be adopted by clinicians.
Potential Impact of QML in The Next Five Years:
Despite these challenges, we believe that QML will have a significant impact on healthcare in the next five years. Quantum hardware is rapidly improving, with new technologies such as superconducting qubits and trapped ions promising to significantly increase the number of qubits and reduce error rates. As quantum hardware improves, researchers will be able to develop and test more complex QML algorithms.
We expect that QML will have a significant impact on drug discovery in the next five years. As more QML algorithms are developed and tested, we expect that they will be integrated into the drug discovery pipeline, accelerating the identification of new drug candidates and reducing the time and cost of drug development. QML algorithms may also be used to predict the toxicity of potential drugs, reducing the risk of adverse effects in clinical trials.
In medical imaging, we expect that QML algorithms will become increasingly important in the next five years. As medical imaging technology improves and produces larger datasets, QML algorithms will be able to identify more subtle patterns in the data and provide more accurate predictions of patient outcomes. QML algorithms may also be used to personalize treatment plans for patients based on their individual imaging data.
Finally, we expect that QML algorithms will be used to develop more accurate predictive models of patient outcomes. QML algorithms can analyze large datasets of patient information and identify patterns that are invisible to classical algorithms. This could lead to more accurate predictions of disease progression and more personalized treatment plans for patients.
Conclusion:
In conclusion, we believe that QML has the potential to transform healthcare in the next five years. Despite the challenges of developing and integrating QML algorithms into clinical workflows, we expect that quantum hardware will continue to improve, enabling researchers to develop more complex QM ..L algorithms. We expect that QML will have a significant impact on drug discovery, medical imaging, and predictive modeling of patient outcomes. As the healthcare industry continues to seek new ways to improve patient outcomes and reduce healthcare costs, we believe that QML will become an increasingly important tool in the healthcare industry. It is prime time to make this happen, and we urge researchers, healthcare providers, and policymakers to collaborate and invest in QML research and development to unlock its full potential in healthcare.
References:
Zeguendry A, Jarir Z, Quafafou M. Quantum Machine Learning: A Review and Case Studies. Entropy (Basel). 2023;25(2):287. Published 2023 Feb 3. doi:10.3390/e25020287
Cordier BA, Sawaya NPD, Guerreschi GG, McWeeney SK. Biology and medicine in the landscape of quantum advantages. J R Soc Interface. 2022;19(196):20220541. doi:10.1098/rsif.2022.0541
Sinha K, Ghosh J, Sil PC. Machine Learning in Drug Metabolism Study [published online ahead of print, 2022 Dec 27]. Curr Drug Metab. 2022;10.2174/1389200224666221227094144. doi:10.2174/1389200224666221227094144
Valdez F, Melin P. A review on quantum computing and deep learning algorithms and their applications [published online ahead of print, 2022 Apr 7]. Soft comput. 2022;1-20. doi:10.1007/s00500-022-07037-4
Cova T, Vitorino C, Ferreira M, Nunes S, Rondon-Villarreal P, Pais A. Artificial Intelligence and Quantum Computing as the Next Pharma Disruptors. Methods Mol Biol. 2022;2390:321-347. doi:10.1007/978-1-0716-1787-8_14