Artificial Intelligence And Machine Learning Algorithms in Prediction of Atrial Fibrillation
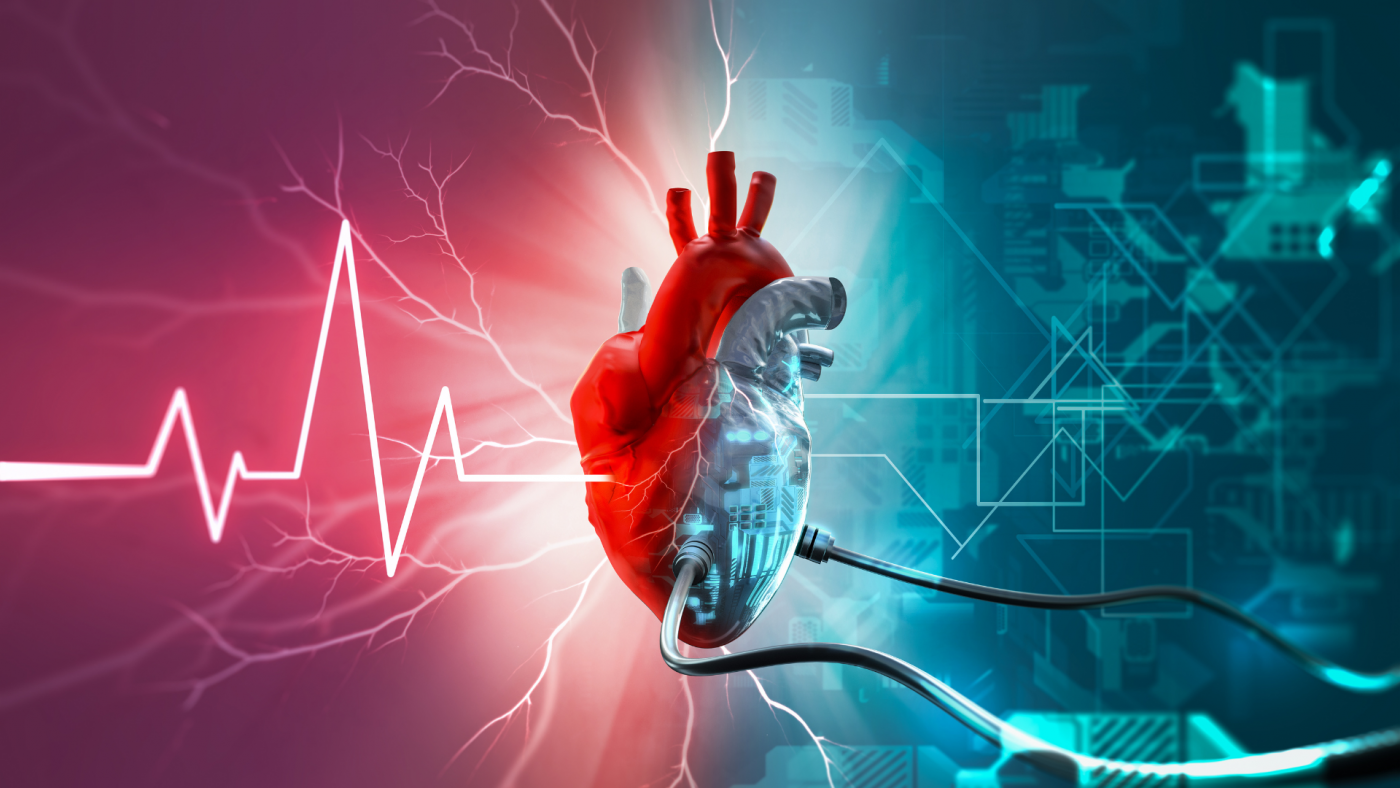
Authors: Amina H Khalpey, PhD, Parker Wilson BS, Brynne Rozell BS, Zain Khalpey, MD, PhD
Atrial fibrillation (AF) is a common type of arrhythmia characterized by rapid and irregular heartbeat, which can lead to serious complications such as stroke and heart failure. Early detection and treatment of AF is crucial for preventing these outcomes, and machine learning algorithms have shown promise in predicting AF using various data sources.
Artificial Intelligence Interpreting ECGs
One approach to using machine learning algorithms to predict AF is through analysis of electrocardiogram (ECG) data. ECG is a non-invasive test that records the electrical activity of the heart and is widely available in clinical settings. ECG data can be used to extract features such as heart rate, heart rate variability (HRV), and the presence of certain waveforms that are characteristic of AF. Using these features, machine learning algorithms can then be developed to identify patterns on ECG that are indicative of AF. One study used a machine learning algorithm to analyze a dataset of ECG recordings from a large cohort of patients with and without AF. The algorithm was able to accurately predict AF with a sensitivity of 87% and a specificity of 84%. Another study used a combination of ECG and demographic data to train a machine learning algorithm to predict AF, and the algorithm achieved a sensitivity of 91% and a specificity of 87%.
AI Can Detect Patterns with Continuous Monitoring Devices
In addition to ECG data, other data sources could be used by machine learning algorithms to predict AF. For example, wearable devices such as smartwatches and fitness trackers can continuously record physiological data such as heart rate and HRV. Devices like these are more accessible outside the clinical setting and could expand the ability to predict AF outside the hospital and clinic. One study used a machine learning algorithm to analyze HRV data collected by a wearable device in a group of patients with AF and a control group. The algorithm was able to accurately predict AF with a sensitivity of 95% and a specificity of 91%. Another study used a machine learning algorithm to analyze a combination of ECG and wearable device data to predict AF, and the algorithm achieved a sensitivity of 96% and a specificity of 89%.
Machine Learning Can Identify Afib Risk Factors
In addition to predicting AF, machine learning algorithms can also be used to identify risk factors for AF. For example, one study used a machine learning algorithm to analyze a combination of clinical, demographic, and ECG data to identify risk factors for AF. The algorithm identified several factors that were significantly associated with an increased risk of AF, including age, hypertension, and diabetes.
The Advantages of AI
There are notable advantages to using machine learning algorithms to predict AF. First, machine learning algorithms can analyze large amounts of data quickly and accurately, which may be difficult for humans to do manually. Second, machine learning algorithms can identify patterns in the data that may not be easily recognizable to humans. Third, machine learning algorithms can be trained to improve their accuracy over time as more data becomes available. Conversely, there are also several challenges to using machine learning algorithms to predict AF. Though algorithms may interpret data quickly, machine learning algorithms also require a large amount of data to be trained, which may not be available in some cases. Second, machine learning algorithms may produce results that are difficult to interpret, which can be a challenge for clinicians. Lastly, machine learning algorithms may be subject to biases in the data, which can affect their accuracy and application. In conclusion, machine learning algorithms have the potential to be a useful and effective tool for predicting AF as well as identifying risk factors for AF. Further research and development is needed to determine the optimal methods for using machine learning algorithms to predict AF and to address the challenges that may arise in their use.