Artificial Intelligence Lung Cancer Screening Platform For Solitary Pulmonary Nodules
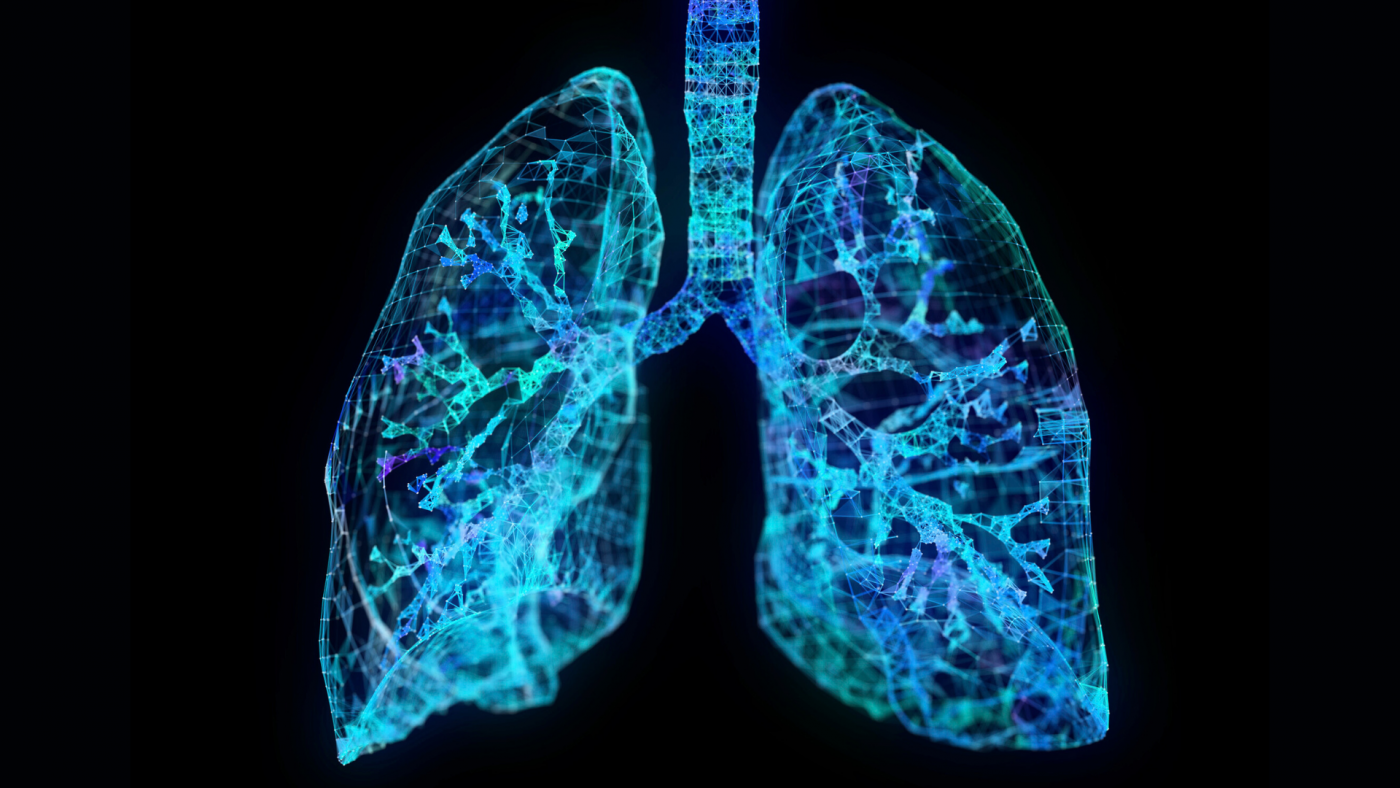
Authors: Amina Khalpey, PhD, Brynne Rozell BS, Zain Khalpey, MD, PhD
Creating a Protocol for AI-Directed Lung Cancer Screening
Lung cancer is one of the leading causes of cancer-related deaths worldwide, with over 1.8 million deaths annually. Early detection is crucial for effective treatment, but traditional screening methods such as chest X-rays and CT scans have limitations in detecting small, solitary pulmonary nodules (SPNs) that may indicate lung cancer. Machine learning algorithms have the potential to improve the accuracy of SPN detection and the prediction of lung cancer. In this blog post, we will discuss the use of machine learning algorithms in predicting lung cancer when screening for SPNs. A 10-step protocol has been created by our team for using CT scans with heat maps to improve the accuracy of SPN detection.
Using Machine Learning to Assess Solitary Pulmonary Nodules
Solitary pulmonary nodules (SPNs) are small, round, or oval-shaped lesions that are less than 3 cm in diameter and are found in the lung. They can be benign or malignant, and the majority of SPNs are benign. However, up to 20% of SPNs are malignant, and they can be difficult to detect on traditional imaging methods such as chest X-rays and CT scans. This is because SPNs are often small and can be difficult to distinguish from surrounding tissue.
Machine learning algorithms have the potential to improve the accuracy of SPN detection and prediction of lung cancer. Machine learning algorithms are computer programs that can learn from data and make predictions or decisions without being explicitly programmed. They have been used in various fields such as image analysis, natural language processing, and medical imaging.
In the context of lung cancer screening, machine learning algorithms can be used to analyze CT scans to identify SPNs and predict whether they are benign or malignant. This can be done by training the algorithm on a dataset of CT scans with known SPN characteristics and outcomes. The algorithm can then be used to analyze new CT scans to identify SPNs and predict their likelihood of being malignant.
10-step Protocol for Using CT Scans with Heat Maps
1. CT scans are obtained for each patient.
2. The CT scans are pre-processed to remove noise and enhance the visibility of SPNs.
3. The CT scans are segmented to identify and isolate SPNs from the surrounding tissue.
4. The characteristics of the SPNs are extracted and used as input for the machine learning algorithm.
5. The machine learning algorithm is trained on a dataset of CT scans with known SPN characteristics and outcomes.
6. The trained algorithm is used to analyze the CT scans obtained in step 1 to identify SPNs and predict their likelihood of being malignant.
7. A heat map is generated to indicate the likelihood of malignancy for each identified SPN.
8. The radiologist reviews the CT scans and the heat map to identify SPNs that require further investigation.
9. Additional imaging or biopsy is performed on suspicious SPNs to confirm their malignancy.
10. The results of the additional imaging or biopsy are used to update the machine learning algorithm and improve its accuracy in the future.
Implementing AI-Cancer Detection
Machine learning algorithms have the potential to improve the accuracy of SPN detection and prediction of lung cancer when screening for solitary pulmonary nodules. The 10-step protocol presented in this post, which combines CT scans with heat maps, provides a systematic approach for using machine learning algorithms in lung cancer screening. Further research is needed to validate the use of machine learning algorithms in clinical practice and to optimize the performance of the algorithm. However, the use of machine learning algorithms in lung cancer screening has the potential to improve the accuracy of SPN detection and ultimately save lives through early detection and treatment of lung cancer.