Health Complications Predicted by Heart Rate Variability and Pulse Oximetry Using Artificial Intelligence
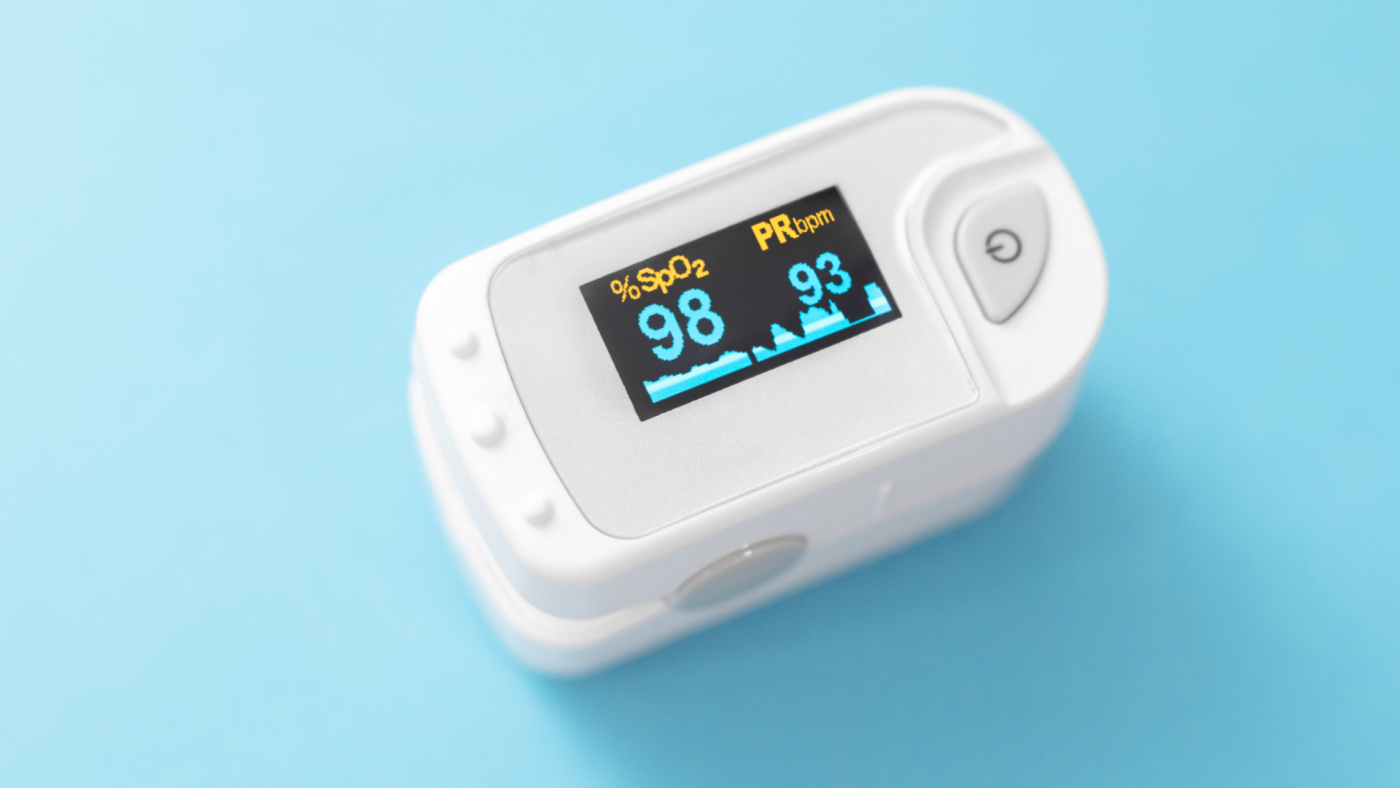
Authors: Amina H Khalpey, PhD, Zain Khalpey, MD, PhD, Brynne Rozell BS, Timothy Ruchti
The evolution of illness leading up to a clinical event is often gradual and entrained across multiple physiological systems. Human observers generally do not have the ability to recognize the time series of patterns within patient measurement data prior to deterioration. Even when these signatures are evident, medical experts capable of identifying them are not available over all time and for all patients. Heart rate variability (HRV) and pulse oximetry are two commonly used medical tests that can provide valuable information about a patient’s cardiovascular and respiratory health. By fusing extracted features from these two measurements, machine learning algorithms have the potential to accurately predict the presence of various cardiac and respiratory pathologies in applied healthcare settings. In this blog post, we will explore the various ways in which machine learning algorithms can be used to predict cardiac and respiratory pathologies by fusing HRV and pulse oximetry data, including:
Early detection of cardiac and respiratory events:
By analyzing HRV and the morphology of the pulse oximetry waveform data in real-time, machine learning algorithms can identify subtle changes that may indicate the presence of a cardiac or pulmonary pathology. This can allow for early intervention and potentially improve patient outcomes.
Personalized treatment recommendations:
Reinforcement learning can be used to generate algorithms that provide personalized treatment recommendations based on a patient’s HRV and pulse oximetry waveform data, taking into account the patient’s unique characteristics and medical history. This can help to optimize treatment and improve patient outcomes.
Risk assessment:
Machine learning algorithms can be used to assess a patient’s risk of developing a particular cardiac or respiratory pathology based on their HRV and pulse oximetry data. This can help to identify patients who may be at high risk for certain conditions and allow for early intervention to prevent or mitigate those risks.
Monitoring treatment response:
Machine learning algorithms can be used to assess the efficacy of therapeutic intervention by analyzing changes in HRV and pulse oximetry data over time. This can help to identify treatment strategies that are most effective for a particular patient and allow for adjustments to the treatment plan as needed.
Predictive modeling:
Machine learning algorithms can be used to build predictive models that can forecast the likelihood of a patient developing a particular cardiac or pulmonary pathology based on their HRV and pulse oximetry data. Such models may be used to continuously evaluate a patient’s status and inform their clinician about the likelihood of a catastrophic event. This can help to identify patients at high risk for certain conditions and allow for early intervention to prevent or mitigate those risks. In conclusion, machine learning algorithms have the potential to significantly improve the accuracy and usefulness of HRV and pulse oximetry in predicting cardiac and pulmonary pathologies in applied healthcare settings. By fusing data from these two tests, machine learning algorithms can enable early detection of pathologies, generate personalized treatment recommendations, assess risk, monitor treatment response, and build predictive models. As machine learning technology continues to advance, it is likely that it will play an increasingly important role in the field of medical diagnosis and treatment.