A White Paper on Machine Learning Improving the Diagnosis and Treatment of Heart and Lung Diseases
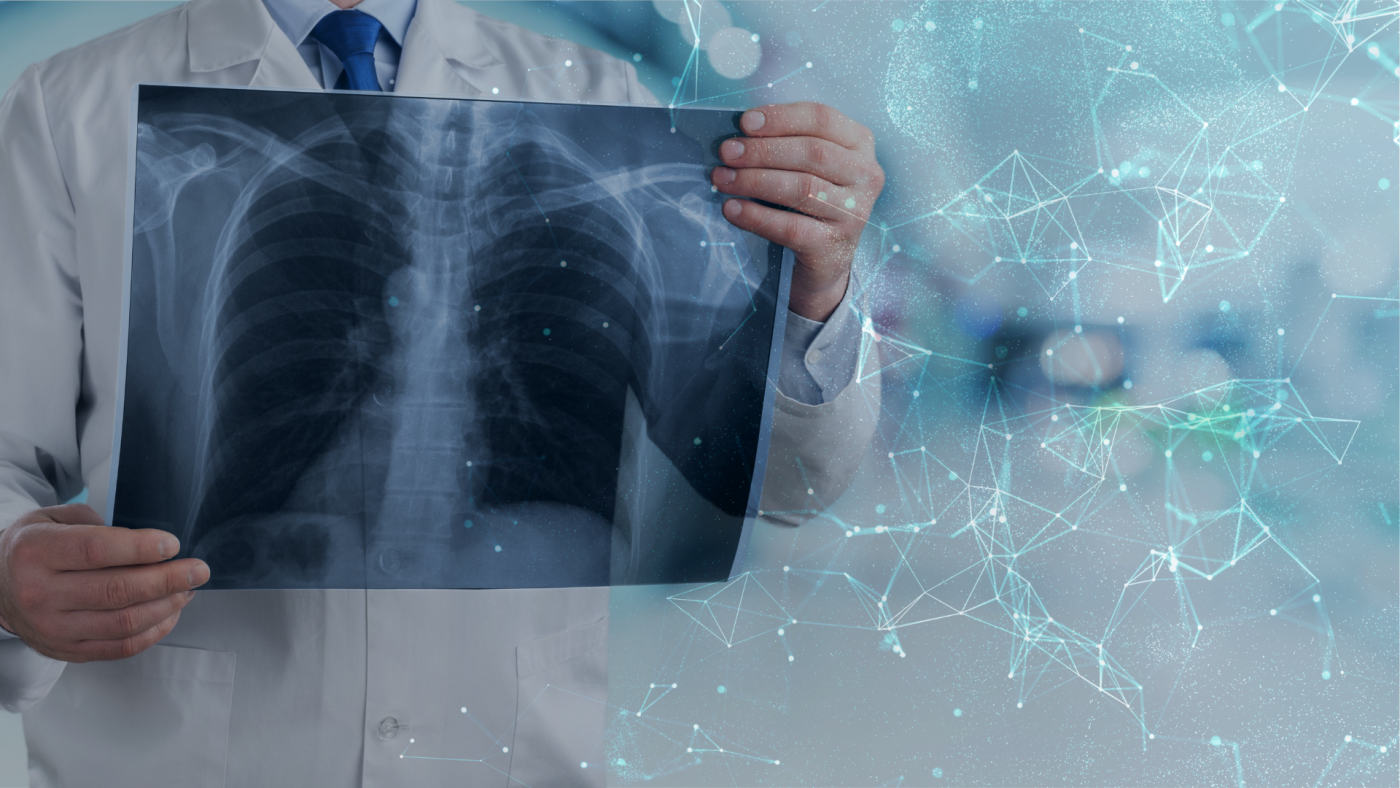
Authors: Amina Khalpey, PhD, Parker Wilson, BS, Zain Khalpey, MD, PhD
Machine Learning (ML) has demonstrated the potential to change the way we provide healthcare. Algorithms and predictive models made with ML could help clinicians immensely in the treatment of heart and lung diseases and potentially reduce healthcare costs, substantially. We believe these algorithms, if developed properly, could be utilized in a wide array of arenas in healthcare including disease detection, diagnosis, treatment and monitoring. With ML, clinicians can analyze vast amounts of data, identify patterns and make better informed decisions more rapidly. By leveraging machine learning in medication management, patients with heart and lung conditions can benefit from personalized treatment plans, better medication adherence, and reduced healthcare costs. This white paper explores the variety of ways machine learning algorithms can improve diagnosis, medical management and monitoring of heart and lung disease patients with specific studies for reference.
Introduction
Heart and lung diseases are among the leading causes of death globally. According to the World Health Organization, cardiovascular diseases (CVDs) account for 17.9 million deaths annually, representing 31% of all global deaths (WHO, 2022). Lung diseases, such as lung cancer and chronic obstructive pulmonary disease (COPD), are also major public health concerns. The diagnosis and anticipation of these diseases is crucial to ensuring the longevity of the patient. Proper anticipatory guidance and testing is often necessary and when the time comes to begin treatment, the right medications should be identified carefully by clinicians. Disease management is typically multimodal, but often involves medications that can be influential in disease progression, or lack thereof. Unfortunately, medical management is often difficult due to patient expense, non-adherence, monitoring and other factors that prevent effective, consistent treatment.
Machine learning (ML) algorithms have the potential to improve all of these arenas of patient care. Algorithms have already been derived to better anticipate disease development and progression using formulae and data already in circulation. Future algorithms are surely to be developed with better, more complete data sets. Medication management could be improved with ML as well by creating personalized treatment plans based on individual patient data. Additionally, ML can rapidly analyze the vast amount of data surrounding medications to best suit patient needs and lifestyle. ML can be used alongside other AI tools to optimize patient-provider communication while monitoring for disease progression and following-up for treatment efficacy. These interventions with ML would hopefully reduce healthcare costs by improving efficiency, analyzing all possible treatment possibilities for patients. This white paper explores the variety of ways ML algorithms can improve diagnosis and treatment of heart and lung disease patients with recent, relevant studies.
Disease Detection and Diagnosis
Early detection and diagnosis of heart and lung diseases is essential for effective treatment and management. Properly trained machine learning algorithms can be utilized to analyze large amounts of patient data, including medical records, lab results, and imaging scans, to more quickly identify patterns and detect early signs of these diseases.
For example, a study published in the Journal of the American College of Cardiology (JACC) demonstrated the use of a ML algorithm for the prediction of mortality and hospitalizations in patients with heart failure with preserved ejection fraction (HFpEF). The algorithm was trained on electronic health record (EHR) data from patients that were a part of the TOPCAT study (2006-2013). Angraal et al (2020) were able to show that their Random Forrest algorithm very nearly matched the real data in prediction of mortality and HF hospitalization; mean concordance-statistics 0.72 + 0.03 and 0.76 + 0.05. This algorithm showed that blood urea nitrogen and Kansas City Cardiomyopathy Questionnaire (KCCQ) were both strong predictors of mortality and hospitalization. If this algorithm were to be used unsupervised or with further data reinforcement, it could feasibly predict both of these outcomes with good accuracy, helping clinicians to remain watchful of their HFpEF patients.
In another example, a study published in the Journal of Thoracic Oncology demonstrated the use of two ML algorithms for the differentiation of small lung nodules to better detect early lung cancer. If caught early, lung cancer, like minimally invasive adenocarcinoma (MIA), can have a 100% 5-year survival, ergo early detection is important. The algorithms were trained retrospectively on computed tomography (CT) scans from over 1,000 patients from multiple centers then evaluated for accuracy in differentiating benign and malignant lesions. Each algorithm was evaluated separately and accuracies varied when comparing different lesions, anywhere from 65% to 90%. Overall, the algorithms improved with more trials through the data and were able to more accurately identify lung nodules that were malignant (Ashraf et al 2021).
By utilizing ML algorithms for disease detection and diagnosis, healthcare providers can identify patients who are at high risk for heart and lung diseases and initiate treatment earlier. This will lead to better health outcomes, lower healthcare costs, and a reduction in the use of expensive medications.
Medication Management
Medication management is a critical component of the treatment and management of heart and lung diseases. However, medication management can be complex and time-consuming, particularly for patients with multiple chronic conditions. Machine learning algorithms can be utilized in medication management to improve medication adherence, reduce the risk of adverse drug events, and lower healthcare costs.
One way that ML algorithms can improve medication management is through the development of personalized treatment plans. By analyzing patient data, including medical history, lab results, and medication usage, ML algorithms can identify patterns and develop personalized treatment plans for each patient that more fluidly integrate into the patient’s lifestyle.
In addition to personalized treatment plans, ML algorithms can also be used to improve medication adherence. Non-adherence to medications is a common problem among patients with chronic conditions, and it can lead to poor health outcomes and increased healthcare costs. ML algorithms can analyze patient data, including medication usage, and develop predictive models to identify patients who are at risk of non-adherence. These patients can then be targeted with interventions to improve their medication adherence, such as reminders or education. Additionally, generative AI could be integrated into applications used by clinicians to build in reminders for patients that have non-adherence tendencies.
A study published in the Journal of Management Care and Specialty Pharmacy tackled this issue with ML. Wirbka et al demonstrated the use of a predictive model for anticoagulant non-adherence in patients with atrial fibrillation. The algorithm was trained on health claim data from over 8,000 patients and was able to accurately predict medication non-adherence with a concordance statistic of 0.755. The study suggested that targeted interventions based on the algorithm’s predictions led to improved medication adherence (Wirbka et al 2022).
ML algorithms can also be used to reduce the risk of adverse drug events (ADEs). ADEs are a common problem among patients taking multiple medications, particularly those with heart and lung conditions. By analyzing patient data, including patient complaints, medication usage and lab results, ML algorithms can identify patients who are at risk of ADEs and develop interventions to reduce the risk. Language processors could be utilized to field common patient complaints and then log them for evaluation of side effects and ADEs.
A study published in the Journal of the American Medical Association (JAMA) demonstrated the use of a ML algorithm to identify patients at high risk of ADEs after opioid dispensation. The algorithm was trained on EHR data from over 800,000 patients and was able to identify some patients at high risk of ADEs, but the study concluded that other probability thresholds may be just as useful. Regardless, if properly stratified, strong data is used to develop these algorithms, ADEs could be anticipated and avoided (Sharma et al 2022).
By utilizing ML algorithms in medication management, healthcare providers can improve medication adherence, reduce the risk of ADEs, and lower healthcare costs.
Monitoring and Follow-Up
Monitoring and follow-up are critical components of the treatment and management of heart and lung diseases. However, both are time-consuming and expensive, particularly for complex patients. ML algorithms can be trained to improve efficiency of monitoring and follow-up and reduce healthcare visits as well as costs.
ML algorithms could improve monitoring through the use of remote monitoring devices. Devices like sphygmomanometers and pulse oximeters could upload patient data to unsupervised algorithms linked to healthcare providers and consistently monitor patients’ vital signs remotely, in the hopes of identifying changes that require follow-up. These algorithms can analyze the data collected then add to predictive models to identify patients who are at risk of hospitalization, disease progression or even mortality.
If a ML algorithm analyzed data collected from remote heart failure monitoring devices like CardioMEMS in patients with heart failure, a cardiologist would reasonably be able to identify patients at risk of volume overload before the patient decompensated and presented to the hospital. If developed properly, the algorithm would be able to accurately predict heart failure exacerbations up to six weeks in advance, allowing for early intervention and improved outcomes.
In addition to remote monitoring, ML algorithms can also be utilized in the analysis of EHR data to identify patients who require follow-up. By analyzing patient data, including medical history and lab results, ML algorithms can develop predictive models to identify patients who are at risk of complications or disease progression. Healthcare providers can then initiate follow-up for these patients to prevent complications and improve health outcomes.
For example, a study published in the Journal of the American College of Cardiology (JACC) demonstrated the use of a ML algorithm to predict readmissions for heart failure patients. The algorithm was trained on EHR data from over 1,900 patients and was able to accurately predict readmissions within the next 90 days with an accuracy of 74% (Park et al 2021). The study suggested that targeted interventions based on the algorithm’s predictions could lead to a reduction in readmissions.
By utilizing ML algorithms in monitoring and follow-up, healthcare providers can improve the efficiency of these processes, prevent complications, and lower healthcare costs.
Cost Savings
The use of ML algorithms in the treatment and management of heart and lung diseases has the potential to significantly reduce healthcare costs. By improving the efficiency and effectiveness of healthcare processes, ML algorithms can reduce the use of expensive medications, prevent complications, and reduce hospitalizations and readmissions.
Algorithms described above in Angraal et al (2020) and Ashraf et al (2022) are just two examples of predictive models and algorithms that could feasibly add to the prognostic power of clinicians in order to better anticipate and treat diseases to hopefully reduce hospitalizations and healthcare costs.
ML algorithms can also help reduce medication costs through personalized treatment plans that account for cost and insurance coverage. Additionally, the pharmacy marketplace is changing drastically with more and more options for patients to find medications at lower prices. Companies like Cost Plus Co and GoodRx have infiltrated an industry that was dominated by only a couple retail pharmacies like CVS and Walgreens. These companies work differently, but both have widened the pharmacy options for patients. ML tools should be devised to find the safest and most cost-effective medical management with a variety of different pharmacies.
Conclusion
The use of ML algorithms in the treatment and management of heart and lung diseases has the potential to significantly reduce healthcare costs. By improving the efficiency and effectiveness of healthcare, ML algorithms have the potential to reduce the use of expensive medications, prevent complications, and reduce hospitalizations and readmissions.
ML algorithms can be utilized in various stages of the healthcare system, including disease detection, diagnosis, treatment, and monitoring. By analyzing large amounts of data, including medical records, lab results, and imaging scans, ML algorithms can identify patterns and detect early signs of these diseases. In medication management, ML algorithms can be used to develop personalized treatment plans, improve medication adherence, and reduce the risk of adverse drug events. In monitoring and follow-up, ML algorithms can improve the efficiency of patient-provider communication, prevent complications, and reduce healthcare costs.
The studies presented in this paper demonstrate the potential of ML algorithms when they are developed properly. It is encouraging to see the potential of these tools to help clinicians find improved ways to anticipate and treat diseases.
However, the implementation of ML algorithms in the healthcare system is not without its challenges. Privacy, security, education and potential bias are all issues that must be addressed.
Despite these challenges, the potential benefits of using ML algorithms in the treatment and management of heart and lung diseases are significant. By leveraging the power of ML algorithms, healthcare providers can improve the quality of care for patients, reduce healthcare costs, and improve health outcomes. As such, the continued development and implementation of ML algorithms in the healthcare system is crucial to the future of healthcare.
References
1. Angraal S, Mortazavi B, Gupta A, et al. Machine Learning Prediction of Mortality and Hospitalization in Heart Failure With Preserved Ejection Fraction. J Am Coll Cardiol HF. 2020 Jan, 8 (1) 12–21.
2. Ashraf S.F., Yin K., Meng C.X., Wang Q., Wang Q., Pu J., et al. Predicting benign, pre-invasive and invasive lung nodules on computed tomography scans using machine learning. J Thorac Cardiovasc Surg. 2022; 163: 1496-1505.e10
3. Park J, Sarijaloo F, Canha C, et al. A HIGH-PERFORMANCE MACHINE LEARNING MODEL TO PREDICT 90-DAY ACUTE HEART FAILURE READMISSION AND DEATH IN HEART FAILURE WITH PRESERVED EJECTION FRACTION. J Am Coll Cardiol. 2021 May, 77 (18_Supplement_1) 783.
4. Sharma V, Kulkarni V, Jess E, et al. Development and Validation of a Machine Learning Model to Estimate Risk of Adverse Outcomes Within 30 Days of Opioid Dispensation. JAMA Netw Open. 2022;5(12):e2248559.
5. Wirbka L, Ruff C, Haefeli WE, Meid AD. A prediction model for nonpersistence or nonadherence to direct oral anticoagulants in hospitalized patients with atrial fibrillation. J Manag Care Spec Pharm. 2022 Oct;28(10):1161-1172.
6. Cardiovascular diseases. World Health Organization. World Health Organization 29.03.2023 fromhttps://www.who.int/health-topics/cardiovascular-diseases#tab=tab_1.