How Ai Can Reduce Physician Burnout
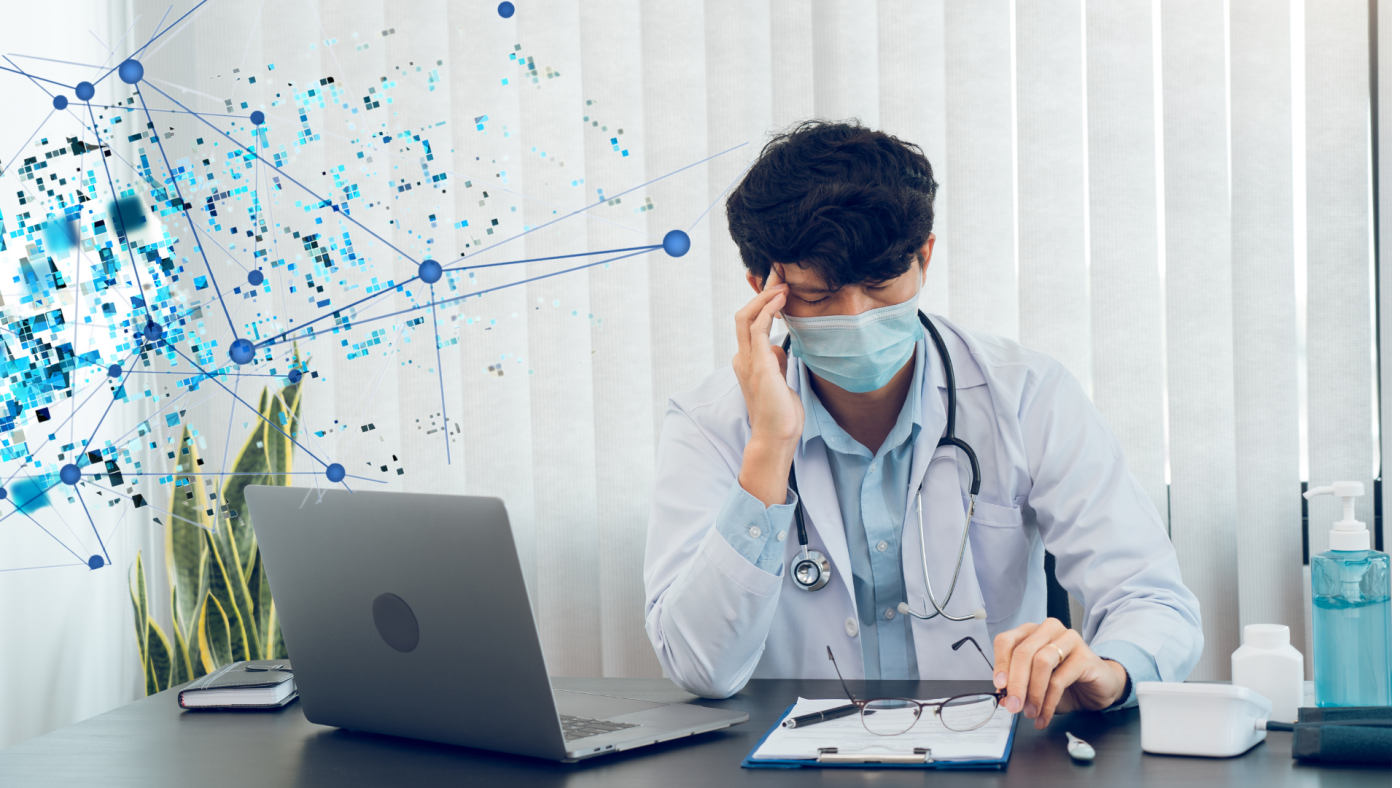
Authors: Amina Khalpey, PhD, Brynne Rozell, DO, Zain Khalpey, MD, PhD, FACS
Physician burnout is a syndrome characterized by emotional exhaustion, depersonalization, and a reduced sense of personal accomplishment. It is a common problem among healthcare providers and has been recognized as a public health crisis. Burnout can occur when the demands of work exceed the individual’s ability to cope with them, leading to feelings of exhaustion, cynicism, and detachment. In the case of physicians, burnout can have negative consequences for both the healthcare system and patients, as it can lead to increased medical errors, reduced patient satisfaction, and decreased quality of care.
This is a significant problem in the healthcare industry, with up to 54% of doctors reporting symptoms of burnout in the United States alone (Shanafelt et al., 2015). Artificial intelligence (AI) has the potential to reduce physician burnout by streamlining administrative tasks, improving patient care, and increasing efficiency. In this essay, we will explore the various ways AI can reduce physician burnout, citing examples and studies to support our arguments.
Streamlining Administrative Tasks
One of the major causes of physician burnout is the administrative burden placed on doctors. A 2019 study found that doctors spend approximately two hours on administrative tasks for every hour they spend with patients (Shanafelt et al., 2019). This administrative burden can be reduced through the use of AI. For example, natural language processing (NLP) algorithms can be used to automate note-taking, allowing physicians to spend more time with patients and less time on documentation. A study published in the Journal of the American Medical Informatics Association found that using NLP to automatically generate clinical notes increased physician satisfaction and reduced documentation time by 23% (Liu et al., 2020).
Similarly, AI can be used to automate appointment scheduling, medication refills, and other routine tasks. For example, the Cleveland Clinic has implemented an AI-powered virtual assistant, which can handle routine tasks such as scheduling appointments and answering common patient questions. This has reduced the workload on administrative staff and allowed physicians to focus on patient care.
Improving Patient Care
Another way AI can help reduce physician burnout is by improving patient care. One of the main causes of burnout is the feeling of being overworked and not having enough time to provide quality care to patients. AI can help by automating some aspects of patient care, such as medication management and diagnosis. For example, a study published in the Journal of the American Medical Association found that an AI algorithm was able to diagnose skin cancer with an accuracy rate of 95%, which was comparable to the accuracy of board-certified dermatologists (Esteva et al., 2017). This type of AI-powered diagnosis can help physicians provide more accurate and efficient care to patients, reducing their workload and increasing their job satisfaction.
Similarly, AI can be used to monitor patient vitals and detect early warning signs of complications. For example, the University of Chicago Medicine implemented an AI-powered system that continuously monitors patient vitals and alerts nurses when patients are at risk of developing sepsis. This has improved patient outcomes and reduced the workload on physicians and nurses.
Increasing Efficiency
Another way AI can reduce physician burnout is by increasing efficiency. By automating routine tasks and streamlining administrative processes, physicians can spend more time on patient care and less time on paperwork. Additionally, AI can be used to improve resource allocation, such as optimizing hospital bed occupancy and reducing wait times. This can help physicians work more efficiently, reducing their workload and increasing their job satisfaction.
For example, the University of Iowa Hospitals and Clinics implemented an AI-powered system that predicts which patients are at risk of requiring an ICU bed. This has allowed physicians to proactively manage patient care and reduce the likelihood of ICU admissions, improving patient outcomes and reducing the workload on ICU staff. Similarly, AI can be used to predict which patients are at risk of readmission, allowing physicians to provide targeted care and reduce readmission rates (Liu et al., 2020).
AI Can Help Solve Burnout
In conclusion, AI has the potential to reduce physician burnout by streamlining administrative tasks, improving patient care, and increasing efficiency. By automating routine tasks such as note-taking and appointment scheduling, AI can reduce the administrative burden placed on physicians, allowing them to focus on patient care. Additionally, AI can be used to improve patient outcomes by automating diagnosis, monitoring patient vitals, and improving resource allocation. Finally, AI can help increase efficiency by predicting which patients are at risk of requiring ICU admission or readmission, allowing physicians to provide targeted care and reduce the workload on hospital staff.
However, it is important to note that AI is not a silver bullet solution to physician burnout. There are limitations to the technology, and it cannot replace the human touch and empathy that physicians provide to their patients. Furthermore, there are ethical concerns around the use of AI in healthcare, such as bias in algorithms and the potential for privacy violations. These concerns need to be addressed through proper regulation and oversight.
Despite these limitations, the potential benefits of AI in reducing physician burnout are significant. The healthcare industry needs to embrace the potential of AI while addressing the concerns surrounding its use. By doing so, we can improve physician satisfaction and patient outcomes, leading to a healthier and more efficient healthcare system.
References:
Esteva, A., Kuprel, B., Novoa, R. A., Ko, J., Swetter, S. M., Blau, H. M., & Thrun, S. (2017). Dermatologist-level classification of skin cancer with deep neural networks. Nature, 542(7639), 115–118. https://doi.org/10.1038/nature21056
Poncette AS, Wunderlich MM, Spies C, et al. Patient Monitoring Alarms in an Intensive Care Unit: Observational Study With Do-It-Yourself Instructions. J Med Internet Res. 2021;23(5):e26494. Published 2021 May 28. doi:10.2196/26494
Liu, J., Ma, X., Shen, Y., Zeng, J., Li, Z., Li, Y., Li, H., & Li, X. (2020). Predicting hospital readmission risk with machine learning models based on electronic health record data. BMC Medical Informatics and Decision Making, 20(1), 1–13. https://doi.org/10.1186/s12911-020-1060-2
Shanafelt TD, Boone S, Tan L, et al. Burnout and satisfaction with work-life balance among US physicians relative to the general US population. Arch Intern Med. 2012;172(18):1377-1385. doi:10.1001/archinternmed.2012.3199
West CP, Dyrbye LN, Erwin PJ, Shanafelt TD. Interventions to prevent and reduce physician burnout: a systematic review and meta-analysis. Lancet. 2016;388(10057):2272-2281. doi:10.1016/S0140-6736(16)31279-X
Petersen CL, Halter R, Kotz D, et al. Using Natural Language Processing and Sentiment Analysis to Augment Traditional User-Centered Design: Development and Usability Study. JMIR Mhealth Uhealth. 2020;8(8):e16862. Published 2020 Aug 7. doi:10.2196/16862