How Can Machine Learning Algorithms For Cardiac Imaging Augment Clinical Decision-Making in The Field?
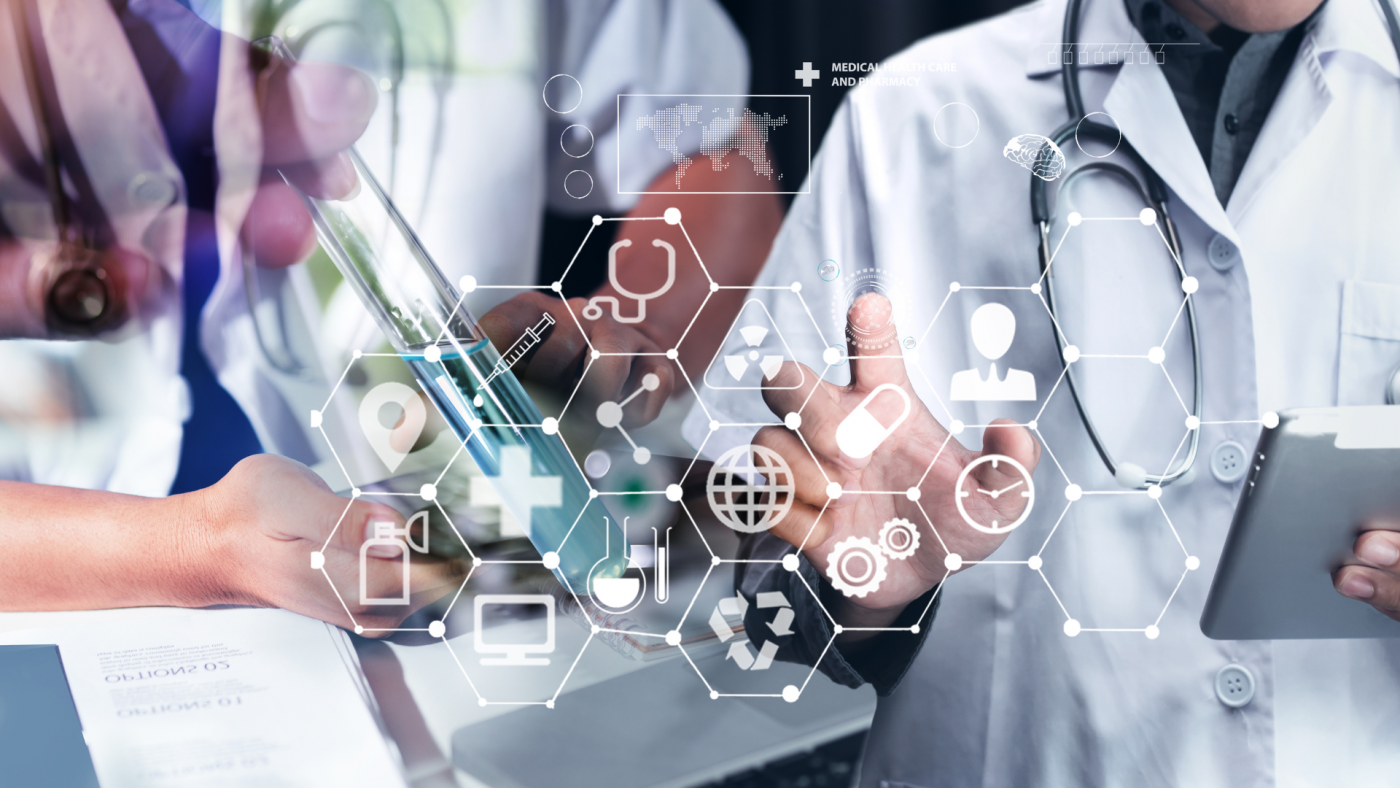
Authors: Amina Khalpey, PhD, Ujjawal Kumar BA, Brynne Rozell BS, Zain Khalpey, MD, PhD
Multimodality cardiac imaging plays a crucial role in the diagnosis, investigation and management of cardiovascular diseases, and machine learning algorithms have the potential to significantly augment clinical decision making in this field. In this blog, we will explore the various areas in which artificial intelligence-machine learning algorithms can be used to improve the accuracy and efficiency of cardiac imaging in the clinical setting, including:
Automated image analysis:
Machine learning algorithms can be used to analyze cardiac imaging scans, such as computed tomography (CT) and magnetic resonance imaging (MRI) scans, to identify abnormalities or potential pathologies. For example, machine learning algorithms can be trained to recognize (on imaging) plaque build-up in the coronary arteries or to identify abnormalities in the heart’s structure or function. This can help to reduce the workload on reporting radiologists and improve the accuracy of diagnoses.
Enhancing image interpretation:
Machine learning algorithms can be used to enhance the interpretation of cardiac imaging scans by providing radiologists with additional information and context. For example, machine learning algorithms can be used to highlight specific areas of the scan that may be of particular interest or to provide relevant clinical data that can help radiologists to make more accurate diagnoses.
Improving image quality:
Machine learning algorithms can be used to improve the quality of cardiac imaging scans by removing noise and artefacts and enhancing the resolution of the images. This can help to improve the accuracy of and confidence in diagnoses and reduce the need for repeat scans, therefore reducing adverse effects on patients such as radiation dose, as well as cost and time.
Predictive modeling:
Machine learning algorithms can be used to build predictive models that can forecast the likelihood of a patient developing a particular cardiovascular condition in the future based on their current imaging scans and other relevant clinical data. This can help to identify patients at high risk for conditions such as heart attack or stroke, allowing for early intervention and potentially improving patient outcomes.
Decision support tools:
Machine learning algorithms can be used to develop decision support tools that can help clinicians to make more informed decisions about a patient’s care. For example, machine learning algorithms can be used to generate personalized treatment recommendations based on a patient’s imaging scans and other data, such as their past medical history and current medications and clinical condition.
In conclusion, machine learning algorithms have the potential to significantly augment decision making in the field of cardiac imaging by automating image analysis, building predictive models, developing decision support tools, enhancing image interpretation, and improving image quality. As machine learning technology and algorithms continue to advance and improve, it is likely that AI-ML will play a constantly increasing role in cardiac imaging, and therefore in the diagnosis and management of cardiovascular diseases.