How Generative AI Can Help Reduce Medical Errors
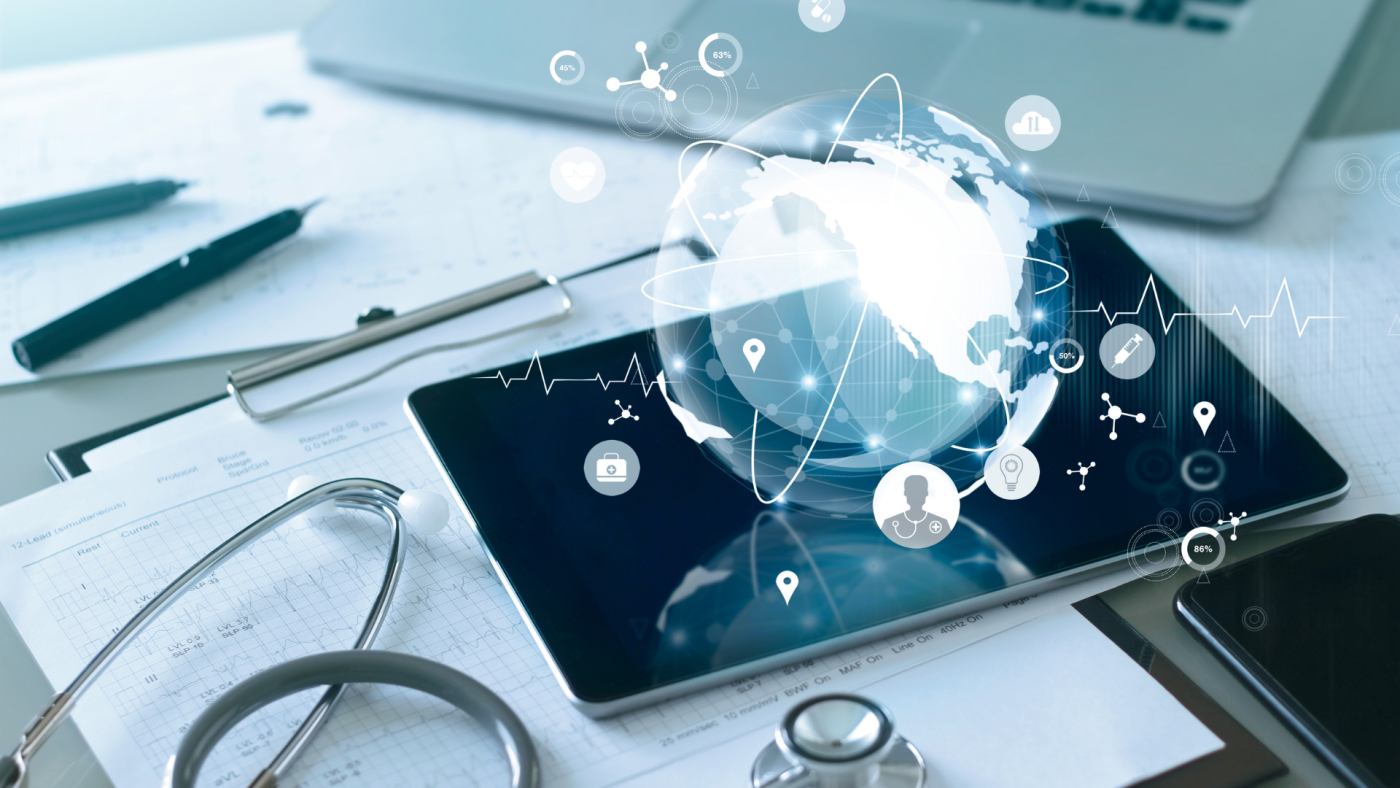
Authors: Amina Khalpey, PhD, Brynne Rozell, DO, Jessa Deckwa, BS, Kirtana Roopan, Zain Khalpey, MD, PhD, FACS
In recent years, there has been a significant increase in the use of Artificial Intelligence (AI) in healthcare. With the advancement in machine learning algorithms, AI has shown potential in the early detection, diagnosis, and treatment of various diseases. One of the areas where AI has shown promising results is in the reduction of medical errors. Medical errors are a significant problem in healthcare and can lead to adverse outcomes, including death. This blog post will explore how generative AI can help the future of healthcare and reduce medical errors.
Generative AI:
Generative AI is a subset of AI that involves the use of algorithms to generate new data or content. This type of AI can learn from the data it is provided and create new data that is similar to it. Generative AI can be used in various applications, including image and speech recognition, text-to-speech conversion, and text generation. In healthcare, generative AI has been used to generate medical images, drug discovery, and electronic health records.
AI models are only as effective as the data on which they are trained. However, these datasets can have class imbalances or sample-selection bias, which affect the accuracy and robustness of the algorithm. Therefore, the use of generative AI to create diverse and representative datasets of the heterogeneity of patient phenotypes and of the equipment and healthcare systems used to collect data (1).
Generative Adversarial Networks (GAN) are a class of machine learning frameworks used in generative AI. They have proved particularly useful in synthetic image generation in healthcare to supply algorithms with more robust datasets upon which they can base medical decisions. GAN utilizes two competing algorithms: a generator component, which synthesizes data, and a discriminator component, which attempts to distinguish synthetic samples from real samples. The generator feeds the images it produces into and learns from the outputs of the discriminator in a feedback loop (2). The result is AI that can create realistic, synthetic data to supplement real data in machine learning.
This framework of AI algorithm has found a wide range of applications in the world of healthcare. Exploration has found potential applications for GAN in ophthalmology by creating new retinal images, molecular oncology by generating positron emission tomography images, and neurology by producing magnetic resonance imaging (5).
Reducing Medical Errors:
Medical errors are a significant problem in healthcare, and they can occur at any stage of the healthcare process, including diagnosis, treatment, and monitoring. According to a report by the National Academies of Sciences, Engineering, and Medicine, medical errors cause an estimated 250,000 deaths in the United States annually. Medical errors are not only costly in terms of human life but also financially. According to a report by the Society of Actuaries, medical errors cost the US healthcare system $19.5 billion annually.
AI has the potential to reduce medical errors in healthcare. Machine learning algorithms can be trained on large datasets of medical data to detect patterns and predict outcomes. These algorithms can assist healthcare providers in making more accurate diagnoses, providing more personalized treatment plans, and reducing the risk of adverse events.
Improving Diagnosis:
One area where AI can reduce medical errors is in the diagnosis of diseases. Diagnostic errors are a significant problem in healthcare and can lead to delayed treatment or the misdiagnosis of a patient. Machine learning algorithms can analyze large datasets of medical data, including electronic health records, medical images, and laboratory results, to identify patterns and predict outcomes. These algorithms can help healthcare providers make more accurate diagnoses, reducing the risk of misdiagnosis or delayed treatment. AI-generated data that mimics the phenotypes of rare diseases and underrepresented patients allows algorithms to make more accurate diagnoses in a broader range of clinical settings.1
In a study published in the journal Nature, researchers developed an AI algorithm that could detect breast cancer in mammograms with high accuracy. The algorithm was trained on a dataset of over 29,000 mammograms and was able to identify cancerous tissue with an accuracy of 94.5%, compared to 89.0% by radiologists. This study demonstrated the potential of AI in improving the accuracy of cancer diagnosis.
Personalized Treatment:
Another area where AI can reduce medical errors is in the development of personalized treatment plans. Traditional treatment plans are often based on population-level data and do not take into account the unique characteristics of each patient. AI can analyze large datasets of medical data to identify patterns and predict outcomes based on individual patient characteristics.
This can help healthcare providers develop personalized treatment plans that are more effective and reduce the risk of adverse events. In a study published in the journal Nature Communications, researchers developed an AI algorithm that could predict the risk of acute kidney injury in critically ill patients. The algorithm was trained on a dataset of over 700,000 electronic health records and was able to predict the risk of acute kidney injury with high accuracy. The study demonstrated the potential of AI in developing personalized treatment plans that are more effective and reduce the risk of adverse events.
Reducing Adverse Events:
AI can also be used to reduce the risk of adverse events in healthcare. Adverse events can occur due to medication errors, incorrect dosage, or delayed treatment. Machine learning algorithms can analyze large datasets of medical data to identify patterns and predict outcomes, which can help healthcare providers identify patients at risk of adverse events and take proactive measures to prevent them.
In a study published in the Journal of Hospital Medicine, researchers developed an AI algorithm that could predict the risk of in-hospital mortality in patients with sepsis. The algorithm was trained on a dataset of over 4,000 patients and was able to predict the risk of in-hospital mortality with high accuracy. The study demonstrated the potential of AI in reducing the risk of adverse events in healthcare by identifying patients at high risk and taking proactive measures to prevent them.
Improving Clinical Documentation:
Another area where AI can reduce medical errors is in the improvement of clinical documentation. Accurate and timely documentation is essential in healthcare, as it can affect the quality of care provided to patients. However, documentation errors, such as incorrect or incomplete information, can lead to medical errors and adverse events.
AI can be used to improve clinical documentation by automating the documentation process and reducing the risk of errors. Natural Language Processing (NLP) is a subset of AI that involves the use of algorithms to analyze and understand human language. NLP can be used to extract information from medical records and generate structured data, which can be used to improve the accuracy and completeness of clinical documentation.
In a study published in the Journal of Medical Internet Research, researchers developed an AI algorithm that could automatically extract clinical information from unstructured medical records. The algorithm was trained on a dataset of over 100,000 medical records and was able to extract information with high accuracy. The study demonstrated the potential of AI in improving clinical documentation by automating the documentation process and reducing the risk of errors.
Information contained in clinical documentation like electronic health records (EHRs) can also be used for research and further AI algorithm development. However, access to routinely collected EHRs is encumbered by ethical and legal issues surrounding unconsented patient data and privacy. Such data is necessary for developing machine learning models. Generative AI algorithms that can synthesize data to supplement that information as well as make the dataset more robust and representative is crucial in training machine learning models that can make sound medical decisions in real-world applications.3
Challenges and Limitations:
Despite the potential benefits of AI in healthcare, there are challenges and limitations that need to be addressed. One of the main challenges is the lack of high-quality data. Machine learning algorithms require large datasets of high-quality data to be trained effectively. However, medical data is often fragmented and incomplete, which can affect the accuracy of AI algorithms.
Recent generative AI algorithms have progressed from synthesizing static patient data to longitudinal EHR time series. This ability is important for assessing risk factors and predicting onset of diseases. While this is an important step, one limitation of such models in making medical decisions is the ability to incorporate data from a variety of sources. In the real-world clinical settings, decisions are made based on information of various types. Modeling this facet of medicine is a challenge that faces generative AI in healthcare (4).
Another challenge is the lack of interpretability of AI algorithms. Machine learning algorithms can be difficult to interpret, and healthcare providers may not trust the results if they cannot understand how the algorithm arrived at a particular decision.
Ethical considerations are also important when using AI in healthcare. For example, there is a risk of bias in AI algorithms, which can lead to unequal access to healthcare for certain groups. Additionally, there is a risk of privacy violations if sensitive medical data is not adequately protected.
Data Labeling:
Data labeling is considered the foundation for machine learning and artificial intelligence algorithm development. Data labeling requires the identification of raw data (i.e., images, text files, videos), and then the addition of one or more labels to that data to specify its context for the models, allowing the machine learning model to make accurate predictions (8). This labeling or cleaning process is similar to preparing a logistic regression data set to which it requires a human in the loop for confirmation of accurate labeling.
Data labeling is a complex and crucial process that plays a significant role in the efficacy of AI in healthcare. By addressing the challenges in data labeling, and embracing a collaborative, patient-centric approach, we can pave the way for AI to significantly improve healthcare delivery and outcomes (9). However, we must remain vigilant about how improper data labeling can affect the accuracy of AI models and lead to a wide variety of medical errors down the line.
Conclusion:
In conclusion, AI has the potential to reduce medical errors in healthcare by improving the accuracy of diagnosis, developing personalized treatment plans, reducing the risk of adverse events, and improving clinical documentation. Generative AI, in particular, can be used to generate new medical data and content, which can be used to improve the accuracy and completeness of medical records and enhance the quality of care provided to patients. However, there are challenges and limitations that need to be addressed, including the lack of high-quality data, the lack of interpretability of AI algorithms, and ethical considerations. As AI continues to advance, it is important to address these challenges and ensure that AI is used in a way that benefits all patients and does not exacerbate existing inequalities in healthcare. With proper implementation and oversight, AI can play a significant role in reducing medical errors and improving the quality of care provided to patients.
References:
1. Chen RJ, Lu MY, Chen TY, Williamson DFK, Mahmood F. Synthetic data in machine learning for medicine and healthcare. Nat Biomed Eng. 2021 Jun;5(6):493-497. doi: 10.1038/s41551-021-00751-8. PMID: 34131324; PMCID: PMC9353344.
2. Arora A, Arora A. Generative adversarial networks and synthetic patient data: current challenges and future perspectives. Future Healthc J. 2022 Jul;9(2):190-193. doi: 10.7861/fhj.2022-0013. PMID: 35928184; PMCID: PMC9345230.
3. Yoon J, Drumright LN, van der Schaar M. Anonymization Through Data Synthesis Using Generative Adversarial Networks (ADS-GAN). IEEE J Biomed Health Inform. 2020 Aug;24(8):2378-2388. doi: 10.1109/JBHI.2020.2980262. Epub 2020 Mar 12. PMID: 32167919.
4. Li J, Cairns BJ, Li J, Zhu T. Generating synthetic mixed-type longitudinal electronic health records for artificial intelligent applications. NPJ Digit Med. 2023 May 27;6(1):98. doi: 10.1038/s41746-023-00834-7. PMID: 37244963; PMCID: PMC10224668.
5. Kazuhiro K, Werner RA, Toriumi F, Javadi MS, Pomper MG, Solnes LB, Verde F, Higuchi T, Rowe SP. Generative Adversarial Networks for the Creation of Realistic Artificial Brain Magnetic Resonance Images. Tomography. 2018 Dec;4(4):159-163. doi: 10.18383/j.tom.2018.00042. PMID: 30588501; PMCID: PMC6299742.
6. Park JE, Vollmuth P, Kim N, Kim HS. Research Highlight: Use of Generative Images Created with Artificial Intelligence for Brain Tumor Imaging. Korean J Radiol. 2022 May;23(5):500-504. doi: 10.3348/kjr.2022.0033. Epub 2022 Apr 4. PMID: 35434978; PMCID: PMC9081688.
7. Cui M, Zhang DY. Artificial intelligence and computational pathology. Lab Invest. 2021 Apr;101(4):412-422. doi: 10.1038/s41374-020-00514-0. Epub 2021 Jan 16. PMID: 33454724; PMCID: PMC7811340.
8. International Business Machines Editorial Content Team. What is data labeling? International Business Machines. Accessed May 24th, 2023. What is Data Labeling? | IBM
9. Khalpey, A., Roopan, K., Deckwa, J., & Khalpey, Z. Medical Mayhem: How Poor Data Labeling is Sabotaging AI in Healthcar. Khalpey AI Lab.