How Generative AI Will Benefit Physicians and Patients
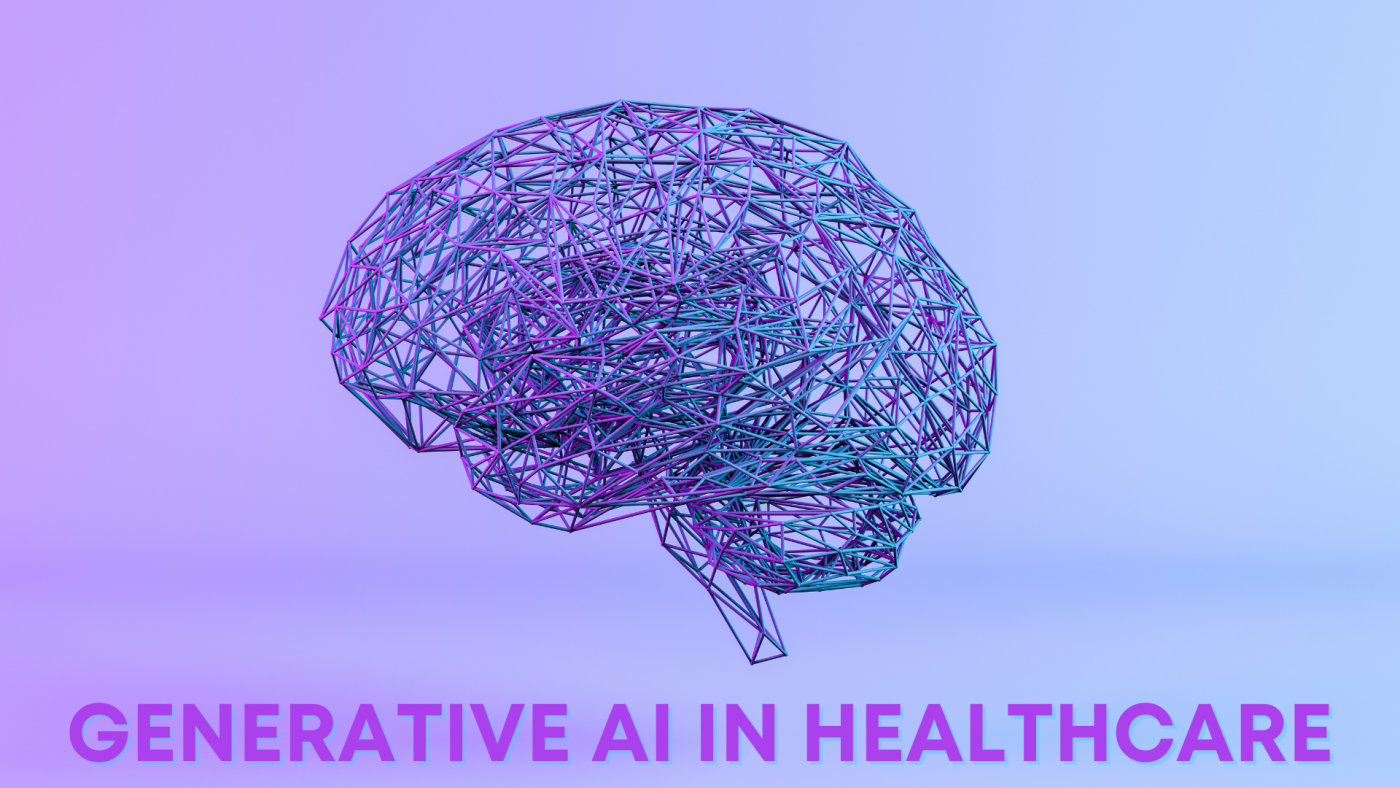
Authors: Amina Khalpey, PhD, Brynne Rozell, BS, Zain Khalpey, MD, PhD, FACS
Generative AI is a branch of Artificial Intelligence that focuses on generating new data or insights based on a given set of input data or criteria. Generative AI techniques such as Generative Adversarial Networks (GANs), Variational Autoencoders (VAEs), and Deep Belief Networks (DBNs) have proven to be extremely useful in a wide range of applications, including image and speech recognition, natural language processing, and drug discovery. In recent years, there has been growing interest in applying generative AI techniques in the field of medicine to improve patient care and outcomes. This blog post explores how Generative AI can be useful to physicians and patients in a non-tech savvy world.
Generative AI in Medical Imaging:
Medical imaging is one area where Generative AI has shown immense promise. Medical images, such as X-rays, CT scans, and MRI scans, are an essential tool for physicians to diagnose and treat various medical conditions. However, the interpretation of medical images is a highly specialized and complex task that requires years of training and experience. Additionally, it is estimated that the field of radiology has an error rate of about 3-5%.
Generative AI techniques can assist physicians in interpreting medical images by automatically identifying and highlighting key features or anomalies in the image that may be indicative of a particular medical condition. For example, GANs can generate realistic synthetic medical images that can be used to train deep learning models to accurately detect and classify various medical conditions. VAEs can be used to generate synthetic images of healthy and diseased organs, which can be used to train medical students and residents to recognize various medical conditions.
Generative AI in Medical Diagnosis:
The medical diagnosis process can also greatly benefit from the information provided by Generative AI. Medical diagnosis involves identifying the underlying cause of a patient’s symptoms and determining the appropriate course of treatment. However, medical diagnosis is often a time-consuming and error-prone process that relies on the physician’s ability to recognize patterns and make accurate diagnoses. Generative AI techniques can assist physicians in making accurate diagnoses by analyzing large amounts of medical data and identifying patterns and relationships that may not be immediately apparent to the physician. For example, DBNs can be used to analyze electronic medical records and identify patients who are at high risk of developing a particular medical condition. GANs can be used to generate synthetic medical data that can be used to train deep learning models to accurately diagnose various medical conditions.
Generative AI in Drug Discovery:
Generative AI can revolutionize drug discovery. Drug discovery is a complex and time-consuming process that involves identifying molecules that can effectively treat a particular medical condition. Traditional drug discovery methods rely on trial and error and are often inefficient and costly. Generative AI techniques can assist in drug discovery by generating new molecules that are predicted to have a specific biological activity or certain drug properties. For example, GANs can be used to generate synthetic molecules that can be screened for potential therapeutic activity. VAEs can be used to generate synthetic molecules that are structurally similar to known drugs, which can be used to identify new drug candidates.
Generative AI in Patient Education:
Patient education is another area where Generative AI can be useful. Patient education involves educating patients about their medical conditions and the appropriate course of treatment. However, patient education is often a time-consuming and difficult task that requires the physician to explain complex medical concepts in a way that the patient can understand. Generative AI techniques can assist the clinician in patient education by generating interactive educational materials that are tailored to the patient’s specific medical condition. For example, GANs can be used to generate interactive 3D models of the human body that can be used to explain various medical conditions and treatments. VAEs can be used to generate patient-friendly summaries of medical research articles that are written in plain language.
Generative AI in Medical Research:
Generative AI also has many applications in medical research. This field of study involves discovering new medical knowledge and developing new treatments for various medical conditions. However, medical research is often a time-consuming and costly process that requires extensive experimentation and analysis. Generative AI techniques can assist in medical research by generating new insights and hypotheses that can be tested and validated through experimentation. This streamlined process frees up researchers to accomplish more meaningful work. For example, DBNs can be used to analyze large amounts of medical data and identify new associations between medical conditions and risk factors. GANs can be used to generate synthetic medical data that can be used to test various hypotheses and theories.
Generative AI in Clinical Trials:
Clinical trials are an essential part of the drug development process. Clinical trials involve testing new drugs or treatments in humans to determine their safety and efficacy. However, these trials are often costly and time-consuming, and many trials fail to produce the desired results. Generative AI techniques can assist in clinical trials by generating synthetic patient data that can be used to simulate the effects of a particular treatment or drug. For example, VAEs can be used to generate synthetic patient data that accurately represents the characteristics of a particular patient population. This can help researchers design more efficient and effective clinical trials that produce more reliable results.
Challenges and Limitations of Generative AI in Medicine:
While Generative AI has shown immense promise in the field of medicine, there are several challenges and limitations that must be addressed. One of the major challenges is the lack of high-quality medical data that is required to train deep learning models. Medical data is often complex, heterogeneous, and highly sensitive, which makes it difficult to collect and share. Additionally, the lack of standardization in medical data collection and storage makes it difficult to compare and integrate data from different sources.
Another challenge is the lack of interpretability and transparency of deep learning models. Deep learning models are often seen as black boxes, which means that it is difficult to understand how the model arrived at a particular conclusion or decision. This lack of transparency can make it difficult to trust the results of deep learning models, particularly in critical medical applications.
Lastly, Generative AI technologies may not be accessible to non-tech-savvy patients and physicians, particularly those in resource-limited areas. This may result in a digital divide where certain patient populations are left behind and unable to benefit from the advancements in Generative AI technology. However, with the proper initiatives and resource allocation, this challenge can be ameliorated.
Generative AI is The Future of Healthcare:
Generative AI has the potential to revolutionize the field of medicine and improve patient care and outcomes. Medical imaging, medical diagnosis, drug discovery, patient education, medical research, and clinical trials are just a few examples of how Generative AI can be useful in the field of medicine. However, there are several challenges and limitations that must be addressed to realize the full potential of Generative AI in medicine. Overcoming these challenges will require collaboration and innovation from researchers, healthcare providers, and policymakers. With the right approach, Generative AI has the potential to transform the way we diagnose and treat medical conditions and improve the health and well-being of patients worldwide.
References:
Maskell G. Error in radiology-where are we now?. Br J Radiol. 2019;92(1095):20180845. doi:10.1259/bjr.20180845
Walters, W.P., Murcko, M. Assessing the impact of generative AI on medicinal chemistry. Nat Biotechnol 38, 143–145 (2020). https://doi.org/10.1038/s41587-020-0418-2
Chen, R.J., Lu, M.Y., Chen, T.Y. et al. Synthetic data in machine learning for medicine and healthcare. Nat Biomed Eng 5, 493–497 (2021). https://doi.org/10.1038/s41551-021-00751-8
Han, C., Rundo, L., Murao, K., Nemoto, T., Nakayama, H. (2020). Bridging the Gap Between AI and Healthcare Sides: Towards Developing Clinically Relevant AI-Powered Diagnosis Systems. In: Maglogiannis, I., Iliadis, L., Pimenidis, E. (eds) Artificial Intelligence Applications and Innovations. AIAI 2020. IFIP Advances in Information and Communication Technology, vol 584. Springer, Cham. https://doi.org/10.1007/978-3-030-49186-4_27
Ghassemi M, Naumann T, Schulam P, Beam AL, Chen IY, Ranganath R. Practical guidance on artificial intelligence for health-care data. Lancet Digit Health. 2019;1(4):e157-e159. doi:10.1016/S2589-7500(19)30084-6
Zhavoronkov A, Vanhaelen Q, Oprea TI. Will Artificial Intelligence for Drug Discovery Impact Clinical Pharmacology?. Clin Pharmacol Ther. 2020;107(4):780-785. doi:10.1002/cpt.1795