How Machine Learning Algorithms Can Augment Precision Delivery of TAVR Valves in Structural Heart Disease
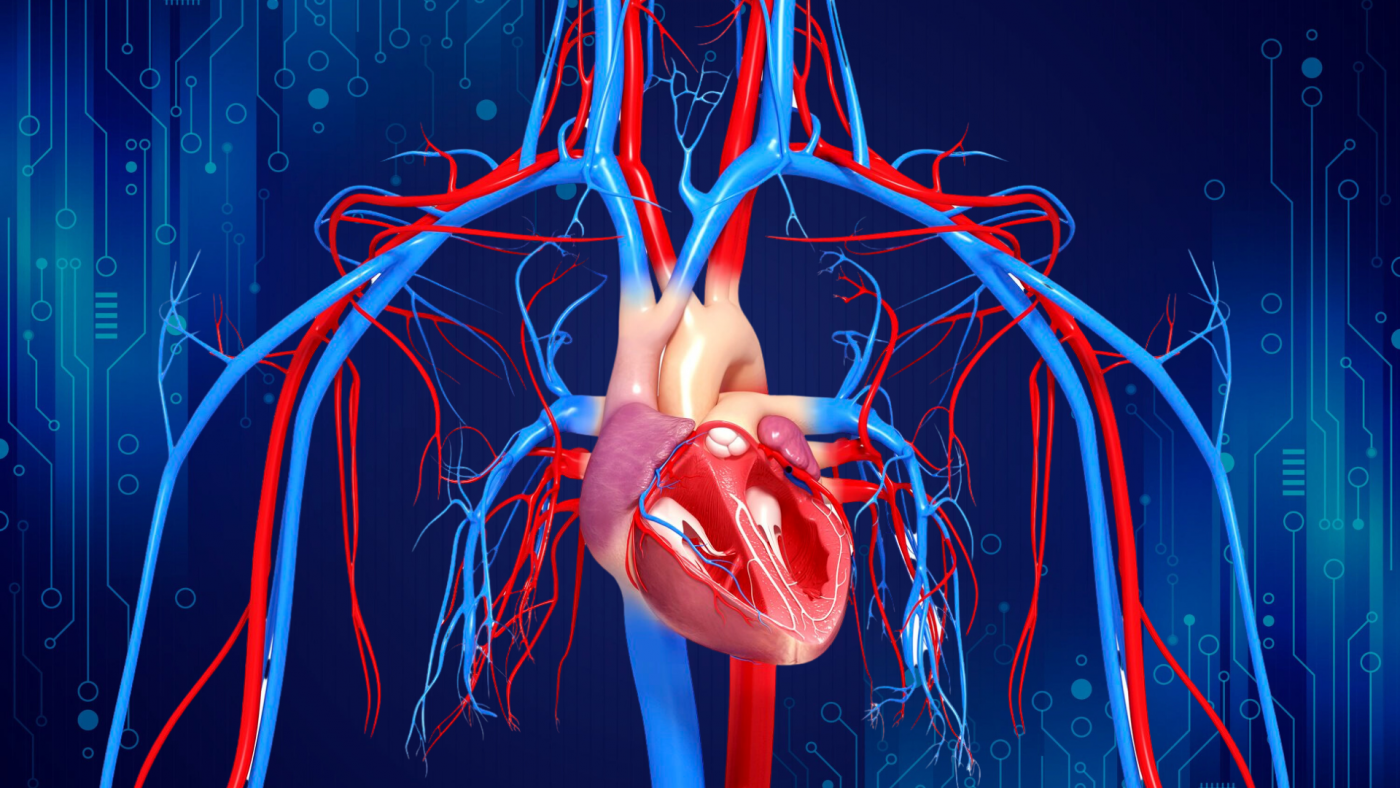
Authors: Zain Khalpey, MD, PhD, FACS, David Rizik, MD, Robert Burke, MD, Robert Riley, MD, Amina Khalpey, PhD, Ujjawal Kumar BA, Brynne Rozell, BS
Transcatheter aortic valve replacement (TAVR) is a minimally invasive procedure for the treatment of severe aortic stenosis in patients who are at high or prohibitive risk for traditional surgical valve replacement. This procedure involves inserting a catheter with a replacement valve through a small incision in the groin or chest and guiding it to the heart through blood vessels.
TAVR has gained popularity in recent years due to its minimally invasive nature, which means it can be done without opening up the chest, leading to less pain, scarring, and a shorter recovery time compared to traditional open-heart surgery. According to a report by the American College of Cardiology, the number of TAVR procedures performed in the United States has increased significantly in recent years. In 2019 alone, there were over 100,000 TAVR procedures performed worldwide.
Machine Learning Can Improve TAVR Precision
TAVR has demonstrated improved outcomes in terms of mortality and morbidity compared to traditional open surgery. However, there is still a significant risk of procedural complications associated with TAVR, including coronary artery obstruction, stroke, and paravalvular regurgitation.
One of the major challenges in TAVR is the precise delivery and deployment of the valve within the aortic annulus. The success of the TAVR procedure depends on accurate preoperative workup and intraoperative delivery, which requires precise imaging and measurements of the aortic annulus and surrounding structures.
Machine learning (ML) algorithms have shown great potential in improving the accuracy of medical imaging analysis and in aiding in clinical decision-making. In this blog post, we will discuss how machine learning algorithms can augment the precision delivery of a transcatheter aortic valve by optimizing the preoperative workup and intraoperative delivery, with a strong focus on imaging protocols.
Preoperative Workup
Preoperative imaging is crucial in identifying the appropriate candidates for TAVR and in selecting the optimal prosthesis size and delivery approach. A variety of imaging modalities are used to assess the aortic valve and surrounding structures, including transthoracic echocardiography (TTE), transesophageal echocardiography (TEE), computed tomography (CT), and magnetic resonance imaging (MRI). Each modality has its strengths and weaknesses, and the choice of modality depends on the clinical scenario and patient-specific factors.
Machine learning algorithms can aid in the analysis of preoperative imaging and in providing more accurate and reliable measurements of the aortic annulus and surrounding structures. For example, ML algorithms can be trained to perform automated segmentation of the aortic valve and annulus, which can facilitate more accurate measurements of annular size and geometry. ML algorithms can also be trained to identify and quantify the extent of calcification, which can impact prosthesis sizing and delivery strategy. ML algorithms can also incorporate the measurements from various imaging modalities together to give clinicians the most accurate structural information.
In addition to providing more accurate measurements, ML algorithms can also aid in the identification of patient-specific risk factors that may impact the success of the TAVR procedure. For example, ML algorithms can be trained to identify patients with a high risk of coronary artery obstruction or paravalvular regurgitation, which may require modifications to the prosthesis sizing or delivery strategy. ML algorithms can also be used to identify patients with an increased risk of stroke, which may require more aggressive anticoagulation or antiplatelet therapy.
Intraoperative Delivery
Intraoperative imaging is essential for guiding the precise delivery and deployment of the TAVR prosthesis. TEE is the primary imaging modality used during TAVR procedures, providing real-time visualization of the aortic valve and surrounding structures. However, TEE has its limitations, including difficulty in accurately assessing the annular dimensions and geometry, and in identifying areas of calcification or aortic dissection.
Machine learning algorithms can help improve the accuracy and reliability of intraoperative imaging during TAVR procedures. For example, ML algorithms can be trained to perform automated registration of preoperative CT or MRI images with intraoperative TEE images, which can provide a more accurate and reliable assessment of annular size and geometry. ML algorithms can also be used to identify areas of calcification or aortic dissection that may not be readily apparent on TEE imaging.
ML algorithms can also aid in real-time decision-making during the TAVR procedure. For example, ML algorithms can be trained to predict the risk of procedural complications based on real-time imaging data and waveform monitors, such as the risk of coronary artery obstruction or paravalvular regurgitation. This information can be used to modify the prosthesis sizing or delivery strategy to minimize the risk of complications and improve procedural outcomes.
ML algorithms can also aid in the selection of the optimal prosthesis size and delivery approach. ML algorithms can be trained to analyze the preoperative imaging data and recommend the optimal prosthesis size and delivery approach based on patient-specific factors, such as annular size, calcification, and aortic geometry. This can improve the accuracy and efficiency of the TAVR procedure, and reduce the risk of complications associated with suboptimal prosthesis sizing or delivery approach.
In addition to augmenting decision-making during the TAVR procedure, ML algorithms can also aid in post-procedural follow-up and monitoring. ML algorithms can be trained to analyze post-procedural imaging data and identify any complications, such as paravalvular regurgitation or stent migration. This can facilitate early detection and intervention, which can improve long-term outcomes and reduce the need for re-intervention.
Challenges and Limitations
Despite the potential benefits of machine learning algorithms in TAVR, there are several challenges and limitations that must be considered. One of the major challenges is the availability and quality of imaging data. Machine learning algorithms require large amounts of high-quality data to be trained effectively. In the case of TAVR, the availability and quality of imaging data may vary depending on the imaging modality used and the patient population. There may also be variability in the interpretation of imaging data, which can impact the accuracy and reliability of machine learning algorithms.
Another challenge is the interpretability of machine learning algorithms. Machine learning algorithms are often considered to be “black box” models, meaning that the internal workings of the model are not readily interpretable. This can make it difficult to understand how the algorithm is making decisions, and to determine the validity of the algorithm’s outputs. In the case of TAVR, it is critical for clinicians to have a clear understanding of how machine learning algorithms are making decisions to ensure that these decisions are clinically appropriate and safe.
Finally, there are regulatory and ethical considerations that must be addressed when implementing machine learning algorithms in clinical practice. There is a need to ensure that machine learning algorithms are rigorously validated and meet the safety and efficacy standards required for medical devices. There is also a need to ensure that the use of machine learning algorithms is transparent and ethical, and that patients are fully informed about the use of these tools in their care
Future Directions
Despite the challenges and limitations of implementing this technology into the TAVR process, the integration of machine learning algorithms into TAVR has the potential to transform the field of interventional cardiology. There are several areas of future research to pursue which may help to advance the use of machine learning algorithms in TAVR.
One area of research is the development of more sophisticated machine learning algorithms that can analyze multiple imaging modalities and provide more comprehensive assessments of the aortic valve and surrounding structures. This may require the integration of different types of imaging data, such as CT, MRI, and TEE, and the development of algorithms that can effectively integrate and analyze these data sources.
Another area of research is the development of machine learning algorithms that can provide real-time guidance during the TAVR procedure. This may require the development of algorithms that can process and analyze large amounts of imaging data in real time, and provide actionable recommendations to the interventional team.
Finally, there is a need for continued research into the safety and efficacy of machine learning algorithms in TAVR. This may involve large-scale clinical trials to validate the use of machine learning algorithms in TAVR, and to assess their impact on procedural outcomes and long-term patient outcomes.
Augmented Clinical Decision-Making and Treatment Delivery
Machine learning algorithms have great potential in improving the accuracy and efficiency of TAVR procedures, particularly in the preoperative workup and intraoperative delivery. ML algorithms can aid in the analysis of preoperative imaging data and in identifying patient-specific risk factors that may impact the success of the TAVR procedure. ML algorithms can also aid in real-time decision-making during the TAVR procedure, and in the selection of the optimal prosthesis size and delivery approach. Finally, ML algorithms can aid in post-procedural follow-up and monitoring, which can facilitate early detection and intervention of complications.
Integrating machine learning algorithms into the TAVR workup and procedure has the potential to greatly improve procedural outcomes, reduce the risk of complications, and improve long-term outcomes for patients with severe aortic stenosis. However, the development and implementation of ML algorithms for TAVR requires extensive validation and testing to ensure the safety and efficacy of these tools. There are also several challenges and limitations that must be addressed, including the availability and quality of imaging data, the interpretability of machine learning algorithms, and regulatory and ethical considerations. Continued research in this area is critical for advancing the field of TAVR and improving the care of patients with severe aortic stenosis.
Continued research in this area is critical for advancing the field of TAVR and improving the care of patients with severe aortic stenosis.
References:
Glenn K. Report finds TAVR is dominant form of aortic valve replacement, outcomes steadily improving in the United States. American College of Cardiology. November 16, 2020.https://www.acc.org/about-acc/press-releases/2020/11/16/18/53/report-finds-tavr-is-dominant-form-of-aortic-valve-replacement-outcomes-steadily-improving-in-the-us.
HUDSON PA, ENG MH, KIM MS, QUAIFE RA, SALCEDO EE, CARROLL JD. A comparison of echocardiographic modalities to guide structural heart disease interventions. Journal of Interventional Cardiology. 2008;21(6):535-546. doi:10.1111/j.1540-8183.2008.00410.x
Cribier A. The development of transcatheter aortic valve replacement (TAVR). Glob Cardiol Sci Pract. 2016;2016(4):e201632. Published 2016 Dec 30. doi:10.21542/gcsp.2016.32 Tee M, Noble JA, Bluemke DA. Imaging techniques for cardiac strain and deformation: comparison of echocardiography, cardiac magnetic resonance and cardiac computed tomography. Expert Rev Cardiovasc Ther. 2013;11(2):221-231. doi:10.1586/erc.12.182
Nasis A, Mottram PM, Cameron JD, Seneviratne SK. Current and evolving clinical applications of multidetector cardiac CT in assessment of structural heart disease. Radiology. 2013;267(1):11-25. doi:10.1148/radiol.13111196
Takagi H, Hari Y, Kawai N, Ando T; ALICE (All-Literature Investigation of Cardiovascular Evidence) Group. Meta-Analysis and Meta-Regression of Transcatheter Aortic Valve Implantation for Pure Native Aortic Regurgitation. Heart Lung Circ. 2020;29(5):729-741. doi:10.1016/j.hlc.2019.04.012