How Machine Learning Algorithms Predict Complications in Congenital Heart Disease
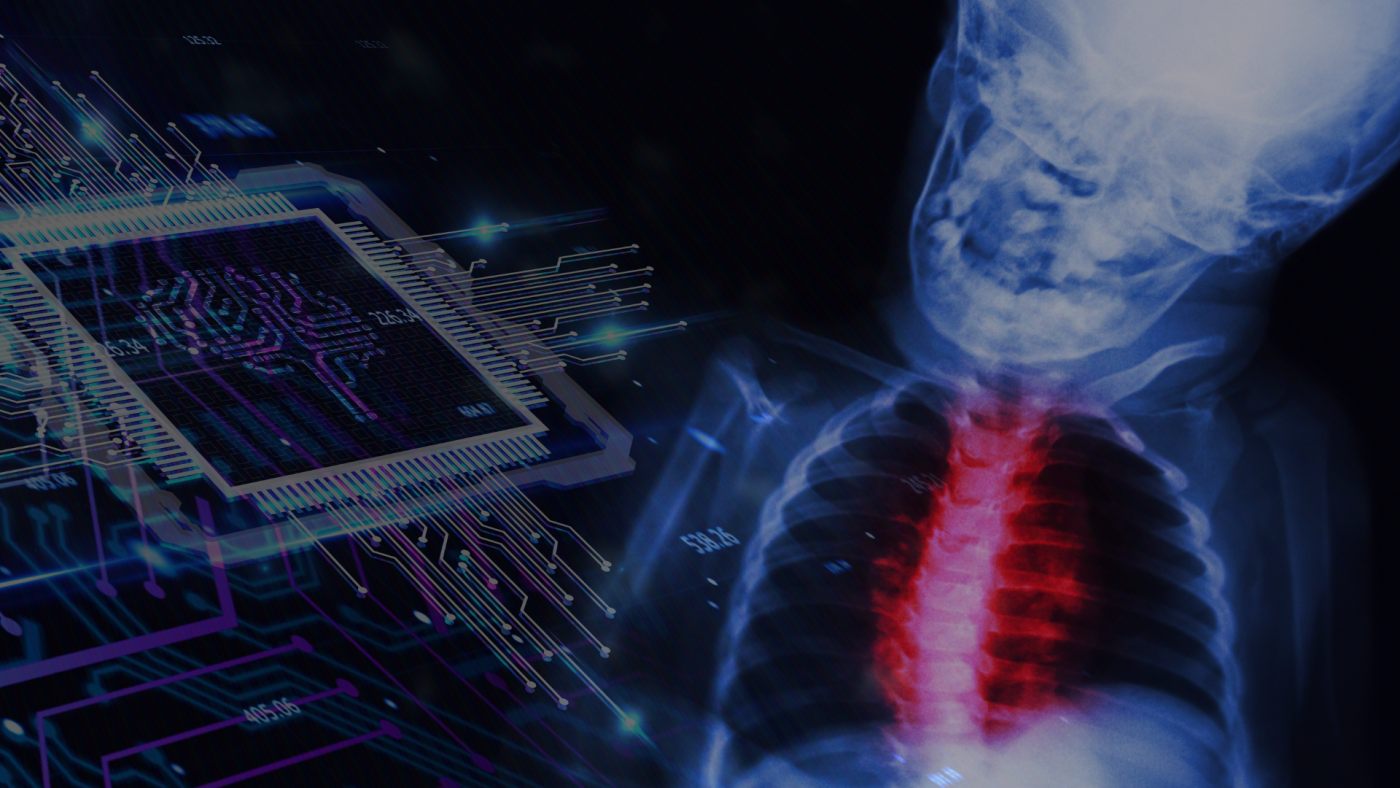
Authors: Amina H Khalpey, PhD, Brynne Rozell BS, Zain Khalpey, MD, PhD
Congenital heart disease (CHD) is a common birth defect that affects the structure of the heart and can lead to serious complications if not properly managed. Machine learning algorithms have the potential to significantly improve the accuracy and reliability of predicting complications in CHD by analyzing large amounts of data and identifying patterns and trends that may not be apparent to human analysts. In this blog post, we will explore the various ways in which machine learning algorithms can be used to predict the complications of CHD, including:
Early detection of complications: Machine learning algorithms can be used to analyze data from a variety of sources, such as medical records, imaging scans, and genetic tests, to identify patterns and trends that may indicate the presence of a complication. This can allow for early intervention and potentially improve patient outcomes.
Risk assessment: Machine learning algorithms can be used to assess a patient’s risk of developing a particular complication based on their medical history, imaging scans, and other relevant data. This can help to identify patients who may be at high risk for certain complications and allow for early intervention to prevent or mitigate those risks.
Personalized treatment recommendations: Machine learning algorithms can be used to generate personalized treatment recommendations based on a patient’s unique characteristics and medical history. This can help to optimize treatment and improve patient outcomes.
Monitoring treatment response: Machine learning algorithms can be used to monitor a patient’s response to treatment by analyzing changes in their medical data over time. This can help to identify treatment strategies that are most effective for a particular patient and allow for adjustments to the treatment plan as needed.
Predictive modeling: Machine learning algorithms can be used to build predictive models that can forecast the likelihood of a patient developing a particular complication based on their medical data. This can help to identify patients at high risk for certain complications and allow for early intervention to prevent or mitigate those risks.
In conclusion, machine learning algorithms have the potential to significantly improve the accuracy and reliability of predicting complications in CHD. By analyzing large amounts of data and identifying patterns and trends, machine learning algorithms can enable early detection of complications, assess risk, generate personalized treatment recommendations, monitor treatment response, and build predictive models. As machine learning technology continues to advance, it is likely that it will play an increasingly important role in the management of CHD.