How Machine Learning Algorithms Will Reduce Medical Errors in Trauma Patients
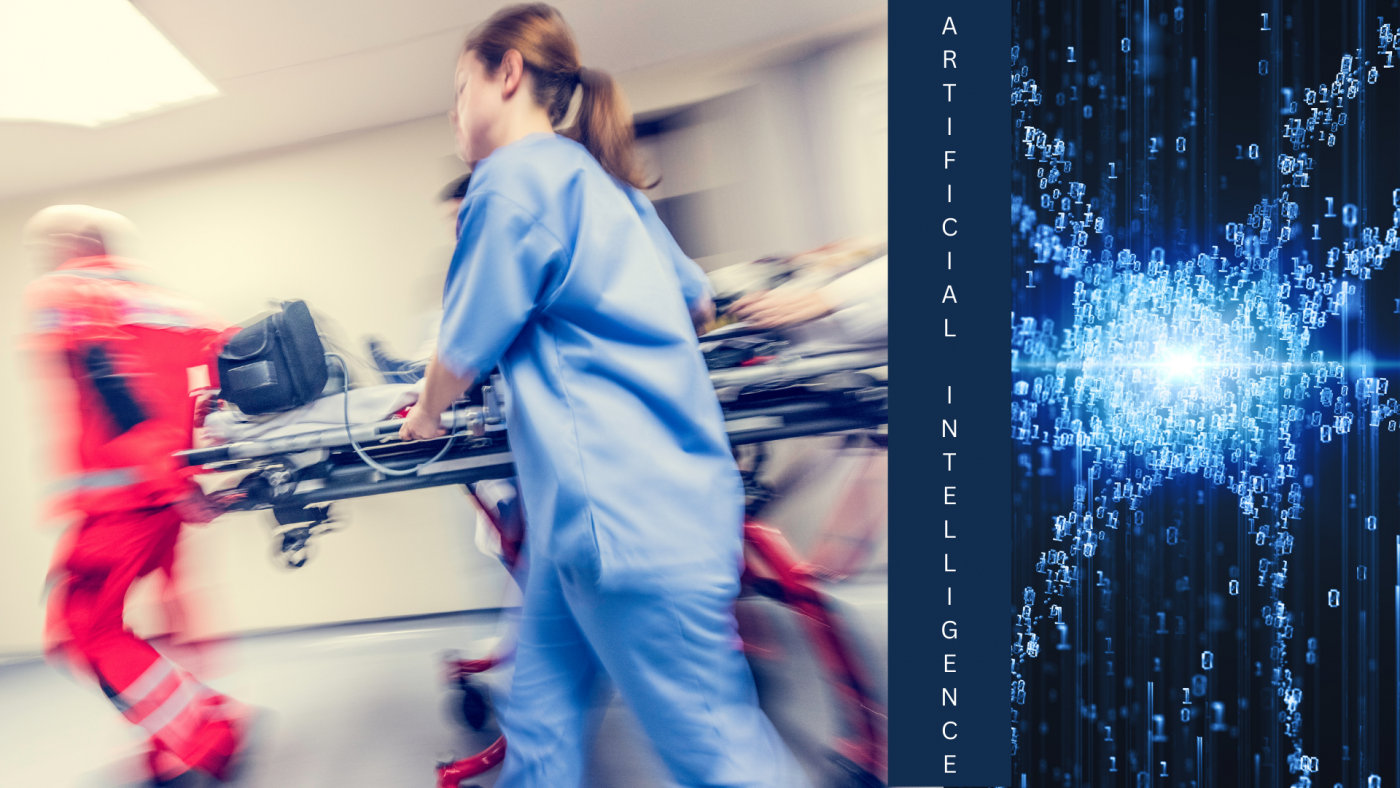
Authors: Amina Khalpey, PhD, Brynne Rozell, BS, Zain Khalpey, MD, PhD, FACS
Medical imaging is a critical component of modern medicine, providing a non-invasive method of diagnosing and monitoring various diseases and pathologies. Imaging is very helpful in identifying critical ailments in trauma emergencies, such as bone fractures and organ injuries. Medical imaging technologies such as X-rays, CT scans, and MRIs are widely used, but they are not infallible. Medical errors, including misdiagnoses, can occur due to a variety of reasons, including human error, technical issues with the imaging equipment, and other factors. These errors can have serious consequences for patients, including delayed or incorrect treatment, unnecessary procedures, and even death. The artificial intelligence strategy called machine learning algorithms have the potential to reduce medical errors and misdiagnoses in trauma by improving the accuracy of medical imaging and providing faster, more accurate diagnoses.
Medical Imaging and Trauma:
Trauma is a major cause of death and disability worldwide. According to the World Health Organization (WHO), injuries are responsible for over 5 million deaths each year. Medical imaging is an important tool in diagnosing and treating trauma, allowing physicians to quickly and accurately identify injuries and plan appropriate treatment. Medical imaging technologies such as x-rays, CT scans, MRIs and ultrasounds are commonly used in emergency trauma bays to help aid diagnosis.
X-rays are often the first imaging tool used in trauma cases, as they are quick, easy, and relatively inexpensive. They can provide information about bone fractures, dislocations, and other injuries. CT scans provide more detailed images than X-rays and can help to identify injuries such as bleeding in the brain, internal organ damage, and other injuries that may not be visible on X-rays. MRIs provide detailed images of soft tissues such as muscles and ligaments and can help to identify injuries that may not be visible on X-rays or CT scans. Ultrasounds are used in bedside FAST exams to immediately identify possible life-threatening conditions in the chest and abdomen.
Medical Errors and Misdiagnoses in Trauma:
Despite the benefits of medical imaging, errors and misdiagnoses can still occur, leading to delayed or incorrect treatment and other more serious sequelae. Some of the factors that can contribute to medical errors and misdiagnoses in trauma include:
Human Error: Radiologists and other healthcare professionals can make errors in interpreting medical images due to factors such as fatigue, distractions, and lack of experience or training. Ultrasounds are especially subjective to user experience and error.
Technical Issues: Problems with the imaging equipment or image quality can lead to errors in interpreting medical images.
Communication Breakdowns: Poor communication between healthcare professionals, including failures to share information about a patient’s medical history or previous imaging studies, can contribute to misdiagnoses.
Time Constraints: Healthcare professionals are under immense pressure to make quick decisions in trauma cases, which can sometimes lead to errors and misdiagnoses.
Machine Learning and Medical Imaging:
Machine learning algorithms have the potential to improve the accuracy and speed of medical imaging, reducing the risk of medical errors and misdiagnoses. Machine learning algorithms are designed to learn from data and can be trained to recognize patterns and make predictions based on input data. In regards to medical imaging, machine learning algorithms can be used to quickly analyze images and identify abnormalities or other factors and patterns that may indicate an injury or condition.
Since machine learning algorithms can be trained using large datasets of medical images, this allows them to learn from a wide variety of cases and improve their accuracy over time. By analyzing patterns in the images, machine learning algorithms can identify subtle differences that may not be apparent to human observers in a hectic trauma bay. Machine learning algorithms can also provide objective, standardized assessments of medical images, reducing the risk of subjective interpretations and errors.
Examples of Machine Learning in Medical Imaging:
There are many examples of machine learning algorithms already being used in medical imaging to improve accuracy and reduce errors. One example is the use of machine learning algorithms to analyze mammograms for the improved detection of breast cancer. Machine learning algorithms can be trained to identify subtle abnormalities in the images that may indicate the presence of cancer, improving the accuracy of breast cancer detection.
Another example is the use of machine learning algorithms in diagnosing lung cancer using CT scans. A study published in the journal Nature Medicine in 2019 found that a machine learning algorithm was able to accurately diagnose lung cancer from CT scans with a higher level of accuracy than experienced radiologists. The algorithm was able to identify patterns in the CT images that were indicative of lung cancer, providing a more accurate and reliable diagnosis.
Machine learning algorithms are also being used to improve the accuracy of MRI scans. A study published in the journal Radiology in 2020 found that a machine learning algorithm was able to accurately identify brain tumors on MRI scans, providing faster and more accurate diagnoses than traditional methods. The algorithm was trained on a large dataset of MRI scans and was able to identify patterns in the images that were indicative of brain tumors.
Machine learning algorithms are also being used to improve the accuracy of X-ray interpretation. A study published in the journal Radiology in 2021 found that a machine learning algorithm was able to accurately diagnose hip fractures on X-rays with a higher level of accuracy than experienced radiologists. The algorithm was able to identify subtle differences in the images that were indicative of a fracture, providing a more accurate and reliable diagnosis.
Benefits of Utilizing Machine Learning in Trauma Imaging:
Machine learning algorithms have the potential to provide numerous benefits in trauma imaging, including:
Improved Accuracy: Machine learning algorithms can provide more accurate and reliable diagnoses by analyzing patterns in medical images that may not be apparent to human observers. Machine learning can also allow for the automation of tasks such as image segmentation and image registration, which can improve the efficiency of ultrasound scans.
Faster Diagnoses: Machine learning algorithms can provide faster diagnoses by analyzing medical images in real-time, reducing the time needed for radiologists to review and interpret the images. This faster detection means that doctors will have greater leadtime to treat dangerous and quickly-deteriorating conditions.
Reduced Errors and Misdiagnoses: By providing objective, standardized assessments of medical images, machine learning algorithms can reduce the risk of subjective interpretations and errors.
Increased Efficiency: Machine learning algorithms can help to increase the efficiency of medical imaging by automating tasks such as image analysis and reporting.
Challenges and Limitations of Machine Learning in Trauma Imaging:
Despite the potential benefits of machine learning in trauma imaging, there are also several challenges and limitations that must be considered. Some of the challenges and limitations include:
Data Quality: Machine learning algorithms require large datasets of high-quality medical images to be trained accurately and effectively. Low-quality or incomplete datasets can lead to inaccurate or unreliable results.
Ethical considerations: Machine learning algorithms can raise ethical considerations related to patient privacy, data security, and bias. We suggest our blog posts discussing how to protect patient privacy in an Ai-driven healthcare system.
Algorithm Transparency: Machine learning algorithms can be complex and difficult to understand, making it challenging for healthcare professionals to interpret their results and understand how they arrived at a particular diagnosis.
Read our article about how to create transparent algorithms here
Integration With Existing Systems: Machine learning algorithms may require new or updated infrastructure and systems to be integrated into existing medical imaging workflows, which can be costly and time-consuming.
Conclusion:
Medical errors and misdiagnoses in trauma can have serious consequences for patients, including delayed or incorrect treatment, unnecessary procedures, and even death. Machine learning algorithms have the potential to reduce medical errors and misdiagnoses in trauma bays by improving the accuracy and speed of medical imaging. By analyzing patterns in medical images, machine learning algorithms can provide more accurate and reliable diagnoses, reducing the risk of errors and misdiagnoses. While there are challenges and limitations to implementing machine learning in trauma imaging, the potential benefits are significant and can lead to improved patient outcomes and more efficient healthcare systems. Further research and development in this field are necessary to realize the full potential of machine learning in medical imaging and trauma diagnosis.