How Machine Learning Can Be Used to Predict and Prevent Teen Depression and Suicide
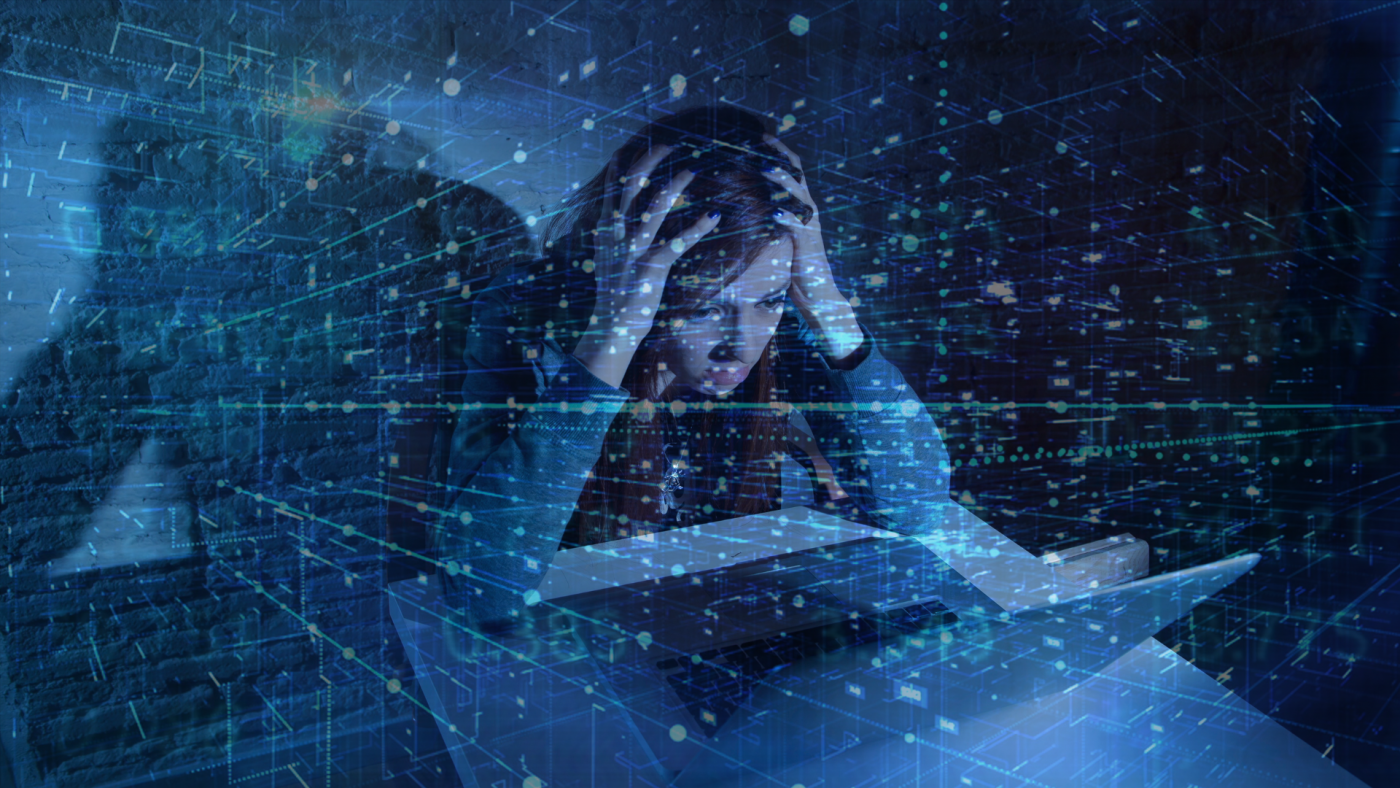
Authors: Amina Khalpey, PhD, Brynne Rozell, BS, Zain Khalpey, MD, PhD
The use of an artificial intelligence tool called machine learning (ML) is a rapidly growing field which has the potential to revolutionize the way we approach mental health issues in patients, especially teenagers. Anxiety, depression, and suicide are among the most pressing mental health concerns facing teens today, and early detection and intervention are crucial for improving outcomes. Machine learning algorithms can be utilized to analyze large amounts of data, including electronic health records, social media activity, and demographic information, to identify patterns and risk factors associated with these conditions. AI tools can be tailored to individual teens, taking into account their unique backgrounds, experiences, and symptoms, which can lead to more personalized and effective interventions. These strategies can also provide a more private and confidential way for teens to access mental health support, which can reduce the stigma associated with seeking help. By utilizing this valuable AI strategy, health professionals will be able to better predict and prevent teen anxiety, depression, and suicide.
How Machine Learning Can Learn and Predict From EHRs:
One of the most promising areas of research in this field is the use of machine learning algorithms to predict teen anxiety, depression, and suicide using electronic health records (EHRs). EHRs contain a wealth of information about an individual’s medical history, including their diagnoses, medications, and laboratory results. Machine learning algorithms can analyze this data to identify patterns and risk factors associated with anxiety, depression, and suicide in teens. For example, research has shown that teens with a history of childhood trauma, family conflict, or a family history of mental illness are at a higher risk of developing these conditions. Machine learning algorithms can identify these teens by analyzing their EHRs, which can then be used to provide targeted interventions and support.
Machine Learning Can Identify Mental Health Problems Through Social Media:
Another promising area of research is the use of machine learning algorithms to predict teen anxiety, depression, and suicide using social media activity. Social media platforms, such as Facebook, Twitter, and Instagram, provide a wealth of information about an individual’s thoughts, feelings, and behaviors. Machine learning algorithms can analyze this data to identify patterns and risk factors associated with anxiety, depression, and suicide in teens. For example, research has shown that teens who express feelings of hopelessness or sadness on social media may be at a higher risk of developing these conditions. Machine learning algorithms can identify these teens by analyzing their social media activity, which can then be used to provide targeted interventions and support such as chatbots and support groups.
Caution Should Be Used in Deploying Machine Learning Technologies:
One of the most important things to consider when using machine learning algorithms to predict and prevent teen anxiety, depression, and suicide is the issue of privacy and ethical concerns. For example, it is important to ensure that the data being used to predict these conditions is collected and analyzed in a way that is compliant with relevant privacy laws and regulations. Additionally, it is important to consider the potential consequences of identifying individuals as being at risk of developing these conditions, such as the possibility of discrimination or other negative outcomes.
Another important thing to consider is that machine learning algorithms are not perfect and may not always provide accurate predictions of anxiety, depression, and suicide in teens. Therefore, it is important to validate the results of any machine learning algorithm used to predict these conditions and to use multiple sources of data to increase the accuracy of predictions. However, since machine learning algorithms are constantly improving based on feedback, these algorithms will continue to improve in accuracy and efficiency.
Using Machine Learning to Identify Teens at Risk and Intervene Early:
In conclusion, machine learning algorithms offer a promising new approach to predicting and preventing teen anxiety, depression, and suicide. By analyzing data from electronic health records, social media activity, and demographic information, machine learning algorithms can identify patterns and risk factors associated with these conditions, providing the potential for early detection and intervention. While there are important ethical and privacy concerns to consider, the potential benefits of using machine learning to improve the mental health of teens make this a promising area of research and development.
References:
Downs J, Velupillai S, George G, et al. Detection of Suicidality in Adolescents with Autism Spectrum Disorders: Developing a Natural Language Processing Approach for Use in Electronic Health Records. AMIA Annu Symp Proc. 2018;2017:641-649. Published 2018 Apr 16.
Hsu JT, Tsai RT. Increased Online Aggression During COVID-19 Lockdowns: Two-Stage Study of Deep Text Mining and Difference-in-Differences Analysis. J Med Internet Res. 2022;24(8):e38776. Published 2022 Aug 9. doi:10.2196/38776
Ueda M, Watanabe K, Sueki H. Emotional Distress During COVID-19 by Mental Health Conditions and Economic Vulnerability: Retrospective Analysis of Survey-Linked Twitter Data With a Semisupervised Machine Learning Algorithm [published correction appears in J Med Internet Res. 2023 Mar 27;25:e47549]. J Med Internet Res. 2023;25:e44965. Published 2023 Mar 16. doi:10.2196/44965
Lopez-Castroman J, Abad-Tortosa D, Cobo Aguilera A, et al. Psychiatric Profiles of eHealth Users Evaluated Using Data Mining Techniques: Cohort Study. JMIR Ment Health. 2021;8(1):e17116. Published 2021 Jan 20. doi:10.2196/17116
Zhou X, Edirippulige S, Bai X, Bambling M. Are online mental health interventions for youth effective? A systematic review. J Telemed Telecare. 2021;27(10):638-666. doi:10.1177/1357633X211047285