Integrating Augmented Artificial Intelligence with Physician Decision-Making: More Precise Cardiovascular Healthcare
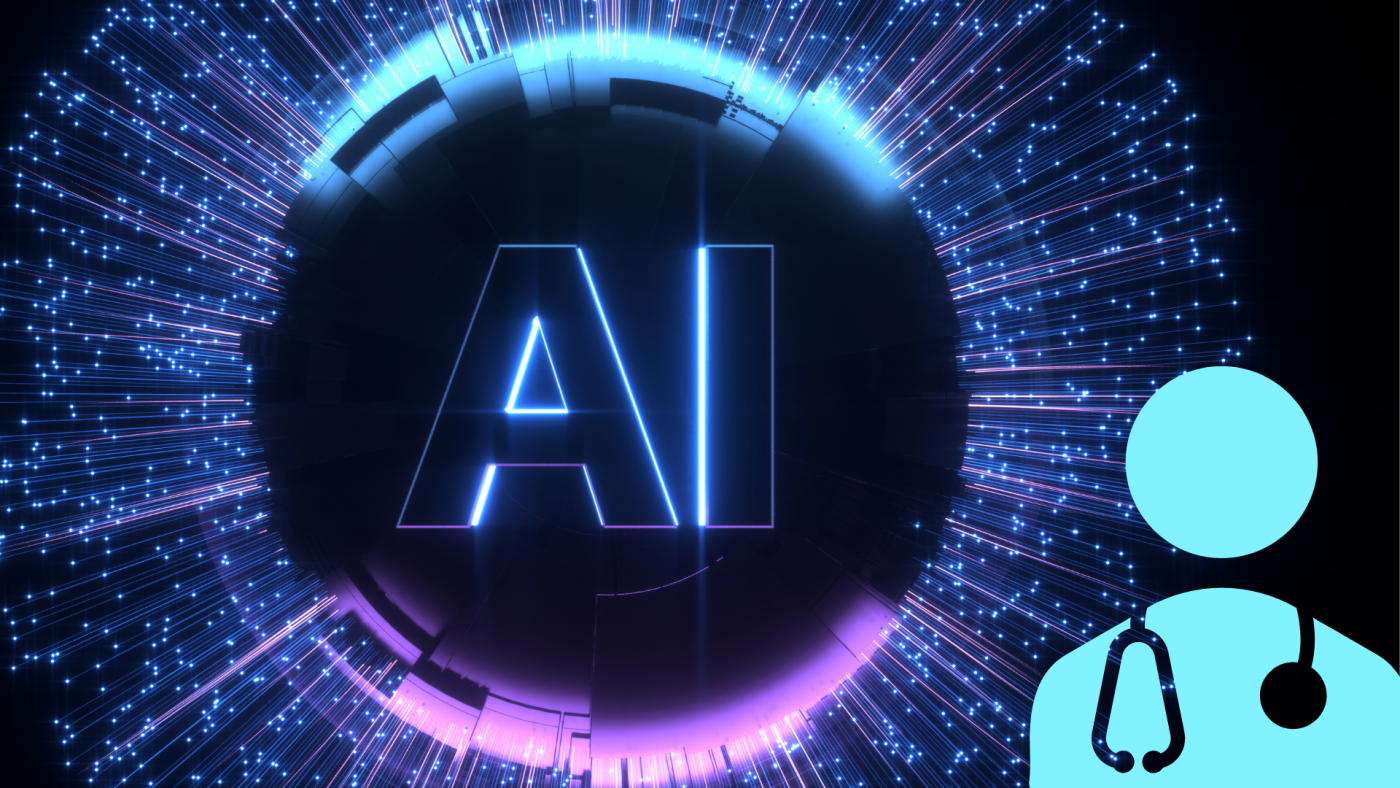
Authors: Amina Khalpey, PhD, Brynne Rozell BS, Zain Khalpey, MD, PhD
Cardiovascular disease (CVD) is a leading cause of morbidity and mortality worldwide. According to the World Health Organization (WHO), CVD accounts for 17.9 million deaths annually, making it the leading cause of death globally. The diagnosis and treatment of CVD have traditionally relied on medical intervention and surgical procedures. However, with the recent advancements in artificial intelligence (AI) technology, there is growing interest in the use of augmented AI for more precise and effective treatment.
Integrating Augmented Ai with Physicians:
Augmented AI refers to the integration of AI with human expertise, where the AI system assists healthcare professionals in decision-making and diagnosis. By leveraging the vast amount of patient data held in EMRs and hospitals, AI can identify patterns and predict outcomes, providing valuable insights to healthcare professionals. In the case of CVD, augmented AI can greatly improve patient care management in the following ways:
Early diagnosis:
CVD often develops silently, making early detection challenging. Augmented AI can help in early diagnosis by analyzing patient data such as electrocardiograms (ECGs), medical images, and patient history. With the assistance of AI, healthcare professionals can identify and treat CVD at its earliest stage, significantly improving patient outcomes.
Personalized treatment:
CVD is a complex condition that requires personalized treatment based on individual patient characteristics. Augmented AI can analyze patient data to identify patterns and predict outcomes, allowing healthcare professionals to develop personalized treatment plans. For instance, AI can analyze patient genetics, lifestyle, and medical history to determine the most effective treatment options.
Predictive modeling:
Predictive modeling is a powerful tool that enables healthcare professionals to identify patients who are at high risk of developing CVD. Augmented AI can analyze patient data and identify risk factors, such as age, gender, and lifestyle, and predict the likelihood of developing CVD. This allows healthcare professionals to develop preventive measures and improve patient outcomes.
Treatment monitoring:
Monitoring patients with CVD is crucial for ensuring effective treatment. Augmented AI can assist healthcare professionals in monitoring patient progress by analyzing patient data such as blood pressure, heart rate, and medical images. This can help in detecting any changes in the patient’s condition and adjusting treatment plans accordingly.
This blog post explores the use of augmented AI in the diagnosis, treatment, and management of CVD. We will begin by examining the current state of CVD treatment and the limitations of traditional methods. We will then explore the benefits and challenges of augmented AI in CVD treatment and discuss the role of AI in early diagnosis, personalized treatment, predictive modeling, and treatment monitoring. Finally, we will discuss the future of augmented AI in CVD treatment and the potential impact on patient outcomes.
Current State of CVD Treatment and Limitations of Traditional Methods
The diagnosis and treatment of CVD has traditionally relied on medical intervention and surgical procedures. Medical intervention includes the use of drugs, lifestyle changes, and interventions to manage risk factors such as hypertension, high cholesterol, and diabetes. Surgical procedures include coronary artery bypass grafting (CABG), angioplasty, and stenting. Despite these treatments, CVD remains a leading cause of death worldwide. Traditional treatment methods have several limitations, including:
Limited predictive ability:
Traditional methods of diagnosis and treatment rely on statistical models and risk factors to predict the likelihood of developing CVD. However, these models have limited predictive ability, and many patients are still diagnosed with CVD despite having low-risk factors.
Ineffective personalized treatment:
CVD is a complex condition that requires personalized treatment based on individual patient characteristics. However, traditional methods of treatment rely on a one-size-fits-all approach, which may not be effective for all patients.
Limited treatment monitoring:
Monitoring patients with CVD is crucial for ensuring effective treatment. However, traditional methods of treatment rely on periodic visits to the physician or hospital for monitoring patient progress. This can lead to delays in detecting any changes in the patient’s condition, which can have a significant impact on patient outcomes.
Limited use of patient data:
Traditional methods of treatment rely on limited patient data, such as medical history, physical examination, and laboratory tests. This can lead to a lack of insight into patient-specific characteristics, which can impact the accuracy of diagnosis and treatment.
Challenges of Integrating Augmented Ai Into Current CVD Treatment:
The use of AI in early diagnosis of CVD has the potential to improve patient outcomes by enabling more effective treatment at the earliest stages of the disease. However, there are challenges associated with the use of AI in early diagnosis. For example, the accuracy of AI algorithms relies on the quality and quantity of patient data used for training the algorithm. In addition, the use of AI in early diagnosis must be accompanied by rigorous evaluation to ensure that the algorithm is effective and accurate. Some other notable challenges include:
Data quality: Augmented AI relies on large amounts of high-quality patient data to provide accurate diagnosis and treatment plans. However, there may be issues with data quality, such as missing data, incorrect data, or biased data, which can impact the accuracy of diagnosis and treatment.
Data privacy: The use of patient data in augmented AI raises concerns about data privacy and security. Healthcare professionals must ensure that patient data is protected and used only for the intended purpose.
Interpretability: AI algorithms can be difficult to interpret, making it challenging for healthcare professionals to understand how the algorithm arrives at a specific diagnosis or treatment plan.
Implementation: The implementation of augmented AI in healthcare settings requires significant investment in technology and infrastructure. Healthcare professionals must be trained to use the technology, and there may be resistance to change from some healthcare professionals.
Role of AI in early diagnosis of CVD
Early diagnosis is crucial in the treatment of CVD. The earlier CVD is diagnosed, the more effective the treatment is likely to be. Augmented Ai can augment early diagnosis by analyzing vast amounts of patient data such as ECGs, medical images, and patient history. AI algorithms can analyze this large amount of data in a short amount of time, which can help identify subtle patterns in patient data that may be missed by human clinicians.
ECGs are a common diagnostic tool used to identify abnormal heart rhythms and detect signs of heart disease. AI algorithms can be trained to analyze ECG data to identify subtle changes in the heart’s electrical activity that may indicate heart disease. These changes may be missed by human clinicians, making the use of AI in ECG analysis a promising tool for early diagnosis of CVD.
Medical imaging, such as echocardiography and cardiac magnetic resonance imaging (MRI), can also provide valuable information for the diagnosis of CVD. Augmented AI can assist in the analysis of medical images, allowing healthcare professionals to identify changes in the heart structure or function that may be indicative of heart disease. AI algorithms can be trained to identify specific patterns in medical images, such as the presence of plaques or the thickening of the arterial walls, which can be early signs of CVD.
The Role of AI in the Personalized Treatment of CVD
CVD is a complex condition that requires personalized treatment based on individual patient characteristics. Augmented AI can help in the development of personalized treatment plans by analyzing patient data to identify patterns and predict outcomes. One area where AI can be particularly helpful is in the development of treatment plans for patients with heart failure. Heart failure is a chronic condition where the heart is unable to pump blood effectively. There are several treatment options for heart failure, including medication, lifestyle changes, and surgical procedures. The choice of treatment depends on several factors, including the patient’s age, medical history, and the severity of the condition. AI algorithms can be trained to analyze patient data to identify the most effective treatment options for individual patients. For instance, AI can analyze patient genetics to determine the best medication for the patient. AI can also analyze patient lifestyle factors, such as diet and exercise, to identify areas where lifestyle changes may improve patient outcomes. Finally, AI can analyze medical history to identify patients who may benefit from surgical procedures.
Ai-Driven Precision Healthcare Can Effectively Improve Patient Outcomes
The use of AI in personalized treatment of CVD has the potential to improve patient outcomes by ensuring that patients receive the most effective treatment for their individual condition. However, there are challenges associated with the use of AI in personalized treatment. The accuracy of AI algorithms depends on the quality and quantity of patient data used for training the algorithm. In addition, the use of AI in personalized treatment must be accompanied by rigorous evaluation to ensure that the algorithm is effective and accurate.
The role of AI in predictive modeling of CVD
Predictive modeling is a powerful tool that enables healthcare professionals to identify patients who are at high risk of developing CVD. Augmented AI can assist in predictive modeling by analyzing patient data and identifying risk factors, such as age, gender, and lifestyle, and predicting the likelihood of developing CVD. AI algorithms can be trained to analyze large amounts of patient data to identify subtle changes that may be indicative of CVD. For instance, AI can analyze patient lifestyle factors, such as diet and exercise, to identify patients who are at high risk of developing CVD. AI can also analyze medical history to identify patients who have a family history of heart disease, which can increase the risk of developing CVD.
The Challenges Facing Predictive Modeling
The use of AI in predictive modeling of CVD has the potential to improve patient outcomes by enabling healthcare professionals to develop preventive measures and identify high-risk patients before the onset of the disease. However, there are challenges associated with the use of AI in predictive modeling. The accuracy of AI algorithms depends on the quality and quantity of patient data used for training the algorithm. In addition, there is a risk of bias in the predictive models if the data used to train the algorithms is not representative of the entire patient population. The use of AI in predictive modeling must be accompanied by rigorous evaluation to ensure that the algorithm is effective and accurate.
Role of AI in Treatment Monitoring of CVD
Monitoring patients with CVD is crucial for ensuring effective treatment. Augmented AI can assist in treatment monitoring by analyzing patient data such as blood pressure, heart rate, and medical images. This can help in detecting any changes in the patient’s condition and adjusting treatment plans accordingly. AI algorithms can be trained to analyze patient data to identify changes in patient condition that may indicate the need for adjustment in the treatment plan. For instance, AI can analyze changes in blood pressure to identify patients who may be at risk of a heart attack or stroke. AI can also analyze medical images to identify changes in the heart structure or function that may be indicative of disease progression. The use of AI in treatment monitoring of CVD has the potential to improve patient outcomes by enabling healthcare professionals to adjust treatment plans as needed.
The Challenges of Ai-Augmented Treatment Monitoring
However, there are challenges associated with the use of AI in treatment monitoring. The accuracy of AI algorithms depends on the quality and quantity of patient data used for training the algorithm. In addition, the use of AI in treatment monitoring must be accompanied by rigorous evaluation to ensure that the algorithm is effective and accurate.
Developing Predictive Risk Prediction Models
One potential application of augmented AI in CVD treatment is in the development of more accurate and personalized risk prediction models. By analyzing large amounts of patient data, AI algorithms can identify subtle changes in patient characteristics that may be indicative of CVD. This can help in the development of more accurate risk prediction models, which can enable healthcare professionals to identify high-risk patients before the onset of the disease.
Cardiovascular disease is a major global health issue and a leading cause of morbidity and mortality worldwide. The treatment of cardiovascular disease has traditionally relied on medical intervention and surgical procedures. However, with the recent advancements in artificial intelligence (AI) technology, there is growing interest in the use of augmented AI for more precise and effective treatment. Augmented AI offers a deeper and more precise approach to treating patients with cardiovascular disease. By leveraging patient data and AI technology, healthcare professionals can improve early diagnosis, personalize treatment plans, predict outcomes, and monitor patient progress more effectively. With the continued advancements in AI technology, it is likely that augmented AI will play an increasingly important role in the treatment of cardiovascular disease and other complex health conditions. However, the implementation of augmented AI in healthcare settings requires significant investment in technology and infrastructure. Healthcare professionals must be trained to use the technology, and there may be resistance to change from some healthcare professionals. Nevertheless, the potential benefits of augmented AI in CVD treatment make it an area of research that is worth exploring further.