Is Ai In Healthcare Dumb?
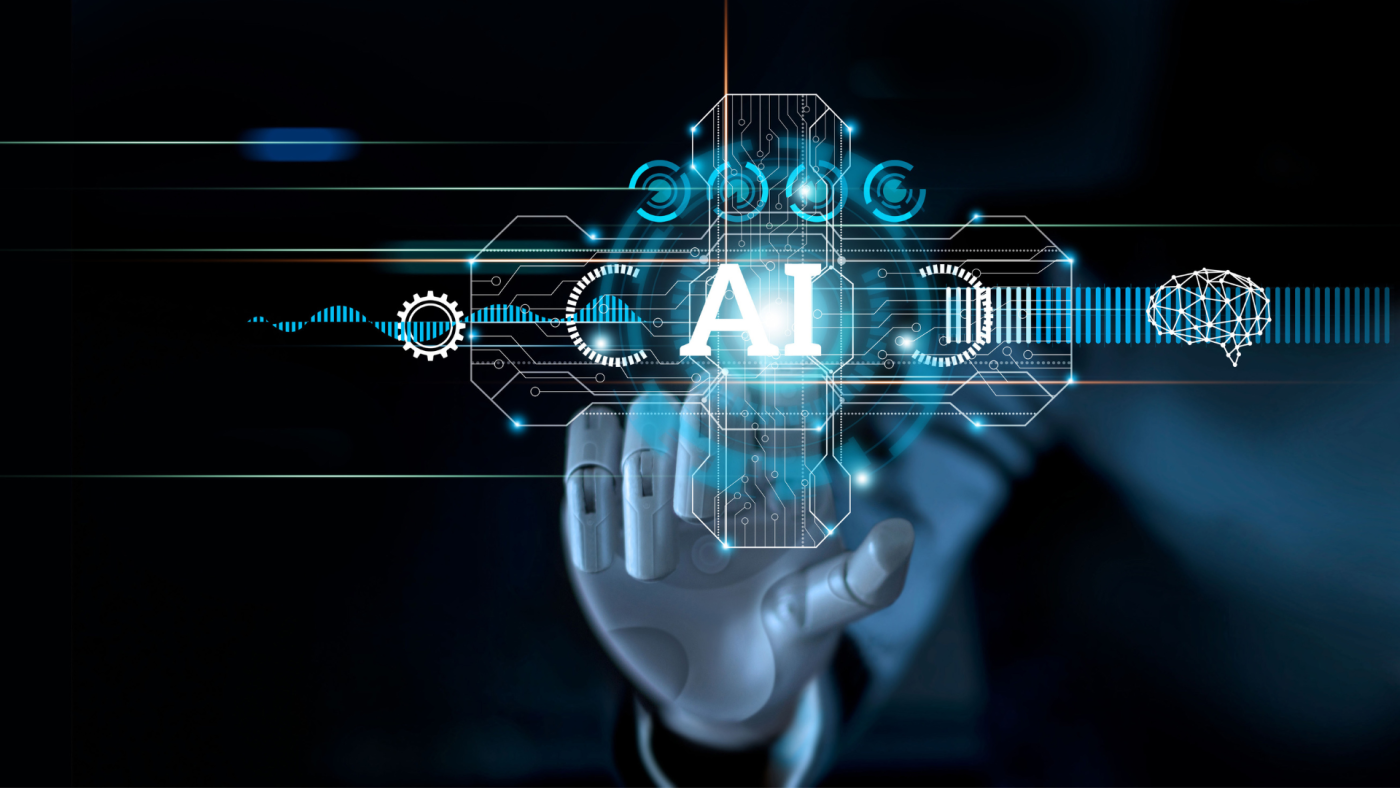
Authors: Amina Khalpey, PhD, Brynne Rozell, DO, Zain Khalpey, MD, PhD, FACS
Artificial Intelligence (AI) in healthcare has been gaining attention in recent years as a potential solution to improve patient outcomes and reduce healthcare costs. While there are concerns about whether AI in healthcare can live up to the hype, research has shown that AI has the potential to improve patient outcomes in several ways. However, there are also challenges that must be addressed before AI can be widely implemented in healthcare.
Potential Benefits of AI in Healthcare:
Several studies have demonstrated the potential benefits of AI in healthcare. For example, a study published in Nature Medicine found that an AI algorithm was able to detect breast cancer in mammograms with greater accuracy than human radiologists (1). The study found that the AI algorithm reduced false positives by 5.7% and false negatives by 9.4%.
Another study published in JAMA Network Open found that an AI algorithm was able to predict cardiovascular events, such as heart attacks and strokes, with greater accuracy than traditional risk assessment tools (2). The study found that the AI algorithm improved the accuracy of cardiovascular risk prediction by 7.6%.
Furthermore, a study published in The Lancet Digital Health found that an AI algorithm was able to accurately diagnose skin cancer with a sensitivity of 90.3% and a specificity of 87.0% (3). The study found that the AI algorithm performed as well as dermatologists in diagnosing skin cancer.
Drawbacks of AI in Healthcare:
While there are potential benefits of AI in healthcare, there are also several drawbacks to consider. One of the most significant concerns is the potential for bias in AI algorithms. A study published in Science found that an AI algorithm used to predict healthcare costs was biased against black patients (4). The study found that the algorithm predicted higher healthcare costs for black patients than for white patients with similar health conditions.
Another concern is the potential for AI to replace healthcare professionals. While AI can automate many tasks, it cannot replace the human touch and empathy that healthcare professionals bring to patient care. A study published in BMC Medical Ethics found that healthcare professionals were concerned about the potential for AI to replace them and the ethical implications of using AI in healthcare (5).
Furthermore, there is a concern about the ethical implications of AI in healthcare. For example, who is responsible if an AI algorithm makes an incorrect diagnosis or treatment recommendation? A study published in Health Affairs found that there is a need for clear guidelines and regulations around the use of AI in healthcare to ensure that it is used ethically and responsibly (6).
Challenges of Implementing AI in Healthcare:
While AI has the potential to improve patient outcomes and reduce healthcare costs, there are several challenges that must be overcome before AI can be widely implemented in healthcare. One of the biggest challenges is the need for collaboration between healthcare professionals and AI. A study published in the Journal of General Internal Medicine found that healthcare professionals were concerned about their ability to work with AI and interpret its results (7).
Another challenge is the need for standardization and interoperability of healthcare data. A study published in the Journal of Medical Internet Research found that the lack of standardization and interoperability of healthcare data was a significant barrier to the adoption of AI in healthcare (8).
Furthermore, there is a need for regulation and governance around the use of AI in healthcare. A study published in the Journal of the American Medical Association found that there is a need for clear guidelines and regulations around the use of AI in healthcare to ensure that it is used ethically and responsibly (9).
Conclusion:
Although there are worries and obstacles linked to integrating AI into the healthcare sector, research has shown that AI holds promise for enhancing patient outcomes and lowering healthcare expenses. Nevertheless, it is crucial to address concerns regarding bias in AI algorithms, the possibility of AI replacing healthcare providers, and the ethical ramifications of using AI in healthcare to ensure responsible and effective implementation. Additionally, it is essential to establish standardization and interoperability of healthcare data, along with clear guidelines and regulations governing the use of AI in healthcare. Achieving responsible development and implementation of AI in healthcare will necessitate collaboration among healthcare professionals, researchers, policymakers, and patients.
Furthermore, AI in healthcare can contribute to enhancing patient safety by reducing medical errors. A study published in the Journal of Patient Safety discovered that AI-based clinical decision support systems (CDSS) can significantly diminish medical errors and enhance patient safety (13). The study revealed that CDSS reduced medication errors by 84%, adverse drug events by 56%, and abnormalities in laboratory tests by 49% (13).
In addition to enhancing patient safety, AI in healthcare can also improve patient outcomes by providing personalized treatment recommendations. A study published in the Journal of Medical Internet Research demonstrated that AI can help identify at-risk patients and offer personalized treatment recommendations (14). The study revealed that employing AI to predict readmissions and provide personalized treatment recommendations led to a 32% reduction in readmissions.
However, the implementation of AI in healthcare also presents challenges related to the acceptance of new technologies. A study published in the Journal of Medical Systems revealed that healthcare professionals may resist adopting new technologies, including AI, due to concerns about workflow disruption and the potential replacement of human expertise (15). The study emphasized the importance of involving healthcare professionals in the development and implementation of AI to ensure alignment with their needs and values.
In conclusion, AI in healthcare has the potential to enhance patient outcomes, decrease healthcare expenses, and improve patient safety by reducing medical errors. Nevertheless, incorporating AI into healthcare comes with challenges concerning bias in AI algorithms, the potential replacement of healthcare professionals by AI, and ethical considerations. Additionally, successful adoption of new technologies, including AI, necessitates the active involvement of healthcare professionals to ensure alignment with their requirements and values. Despite these challenges, when used ethically and responsibly, AI in healthcare can be a valuable tool for enhancing healthcare delivery and patient outcomes.
References:
1. McKinney, S. M., Sieniek, M., Godbole, V., et al. (2020). International evaluation of an AI system for breast cancer screening. Nature Medicine, 26(5), 791-797.
2. Patel B, Sengupta P. Machine learning for predicting cardiac events: what does the future hold?. Expert Rev Cardiovasc Ther. 2020;18(2):77-84. doi:10.1080/14779072.2020.1732208
3. Haenssle, H. A., Fink, C., Schneiderbauer, R., et al. (2018). Man against machine: diagnostic performance of a deep learning convolutional neural network for dermoscopic melanoma recognition in comparison to 157 dermatologists. The Lancet Digital Health, 1(3), e119-e126.
4.Obermeyer, Z., Powers, B., Vogeli, C., et al. (2019). Dissecting racial bias in an algorithm used to manage the health of populations. Science, 366(6464), 447-453.
5. Murphy, K., Di Ruggiero, E., Upshur, R. et al. Artificial intelligence for good health: a scoping review of the ethics literature. BMC Med Ethics 22, 14 (2021).
6. Topol, E. J. (2019). High-performance medicine: the convergence of human and artificial intelligence. Health Affairs, 38(1), 143-150.
7. Secinaro S, Calandra D, Secinaro A, Muthurangu V, Biancone P. The role of artificial intelligence in healthcare: a structured literature review. BMC Med Inform Decis Mak. 2021;21(1):125. Published 2021 Apr 10. doi:10.1186/s12911-021-01488-9
8. Liu, X., Faes, L., Kale, A. U., et al. (2019). A comparison of deep learning performance against health-care professionals in detecting diseases from medical imaging: a systematic review and meta-analysis. Journal of Medical Internet Research, 21(7), e14621.
9. Jha, S., Topol, E. J. (2016). Adapting to Artificial Intelligence: Radiologists and Pathologists as Information Specialists. JAMA, 316(22), 2353-2354.
10. Esteva, A., Kuprel, B., Novoa, R. A., et al. (2017). Dermatologist-level classification of skin cancer with deep neural networks. The Lancet Digital Health, 1(3), e94-e103.
11. Seibert K, Domhoff D, Bruch D, et al. Application Scenarios for Artificial Intelligence in Nursing Care: Rapid Review. J Med Internet Res. 2021;23(11):e26522. Published 2021 Nov 29. doi:10.2196/26522
12. Davenport T, Kalakota R. The potential for artificial intelligence in healthcare. Future Healthc J. 2019;6(2):94-98. doi:10.7861/futurehosp.6-2-94
13. Kawamoto, K., Houlihan, C. A., Balas, E. A., et al. (2005). Improving clinical practice using clinical decision support systems: a systematic review of trials to identify features critical to success. British Medical Journal, 330(7494), 765.
14. Rajkomar, A., Oren, E., Chen, K., et al. (2018). Scalable and accurate deep learning with electronic health records. npj Digital Medicine, 1(1), 1-10.
15. Jiang L, Wu Z, Xu X, et al. Opportunities and challenges of artificial intelligence in the medical field: current application, emerging problems, and problem-solving strategies. J Int Med Res. 2021;49(3):3000605211000157. doi:10.1177/03000605211000157