Is There a Role for Artificial Intelligence in Integrative Healthcare Today?
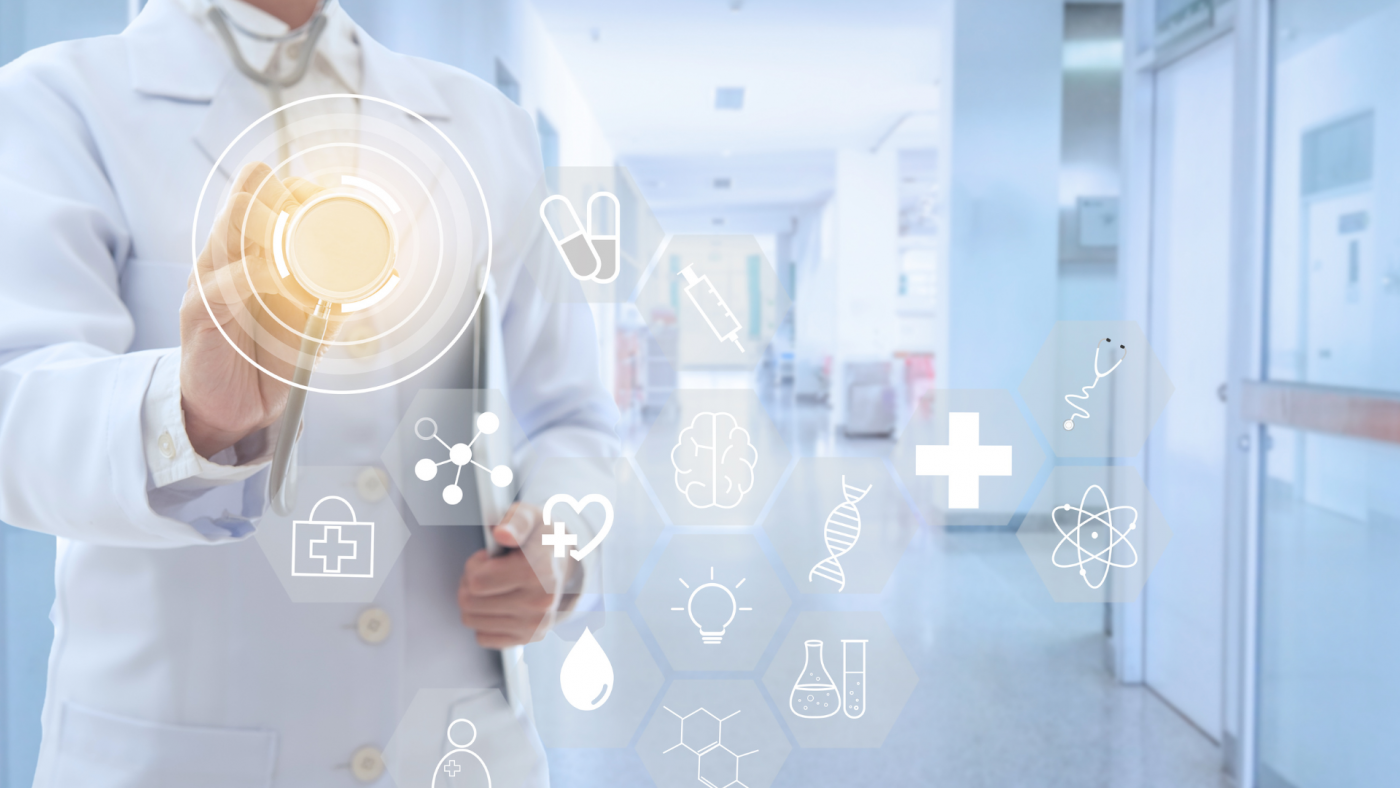
Authors: Amina Khalpey, PhD, Jessa Deckwa, BS, Zain Khalpey, MD, PhD, FACS
Integrative healthcare is a patient-centered approach that combines conventional medicine with complementary therapies to promote healing and well-being. Integrative healthcare recognizes that health is not merely the absence of disease but a state of balance and harmony in the body, mind, and spirit. It also acknowledges that different people have different healthcare needs and preferences, and therefore requires a personalized approach to care.
Machine learning, on the other hand, is a branch of artificial intelligence that enables computers to learn from data without being explicitly programmed. It uses algorithms to identify patterns and relationships in data and makes predictions or decisions based on the patterns it has learned. Machine learning has been used in various applications, including healthcare, to improve patient outcomes, reduce healthcare costs, and increase efficiency.
This blog post will explore the role of machine learning in integrative healthcare. Specifically, it will discuss how machine learning can be used to personalize care, improve diagnosis and treatment, and enhance patient outcomes in integrative healthcare.
Personalized Care:
Personalized care is at the heart of integrative healthcare. It involves tailoring treatment to an individual’s unique needs, preferences, and circumstances. Machine learning can be used to personalize care by analyzing large amounts of data, including patient medical history, genetic information, lifestyle, and social determinants of health, to create individualized treatment plans.
For example, machine learning can be used to identify patterns in patient data that suggest an increased risk of developing a particular condition or disease. Machine learning is diverse in application which can make real-time decisions based on one differing input from an observed pattern. Or by analyzing data from multiple sources, including electronic health records (EHRs), wearable devices, and genetic testing, machine learning algorithms can predict an individual’s risk of developing a disease with a high degree of accuracy. This information can then be used to create a personalized prevention plan that includes lifestyle changes, screening tests, and other interventions that are tailored to the individual’s specific needs.
Machine learning can also be used to personalize treatment by analyzing data on the effectiveness of different therapies for different patients. By identifying patterns in patient data, such as medical history, demographic information, and treatment response, machine learning algorithms can predict which treatments are most likely to be effective for a particular individual. This information can then be used to develop a personalized treatment plan that maximizes the chances of success and minimizes side effects.
Improving Diagnosis and Treatment:
Machine learning can also be used to improve diagnosis and treatment in integrative healthcare. By analyzing large amounts of data from multiple sources, including EHRs, lab results, and medical imaging, machine learning algorithms can identify patterns and relationships that are not immediately apparent to healthcare providers. This can lead to more accurate and timely diagnosis and treatment of conditions and diseases.
For example, machine learning can be used to analyze medical images, such as MRI and CT scans, to detect abnormalities that might be missed by human observers. By comparing a patient’s medical image to a database of thousands of images, machine learning algorithms can identify subtle patterns that indicate the presence of a disease or condition. This can help healthcare providers make more accurate diagnoses and develop more effective treatment plans.
Machine learning can also be used to improve the effectiveness of conventional therapies by identifying patterns in patient data that suggest a need for complementary therapies. For example, machine learning algorithms can analyze EHRs and other data sources to identify patients who are not responding well to conventional treatments for a particular condition. By analyzing data on the effectiveness of complementary therapies for similar patients, machine learning algorithms can predict which complementary therapies are most likely to be effective for the individual in question. This information can then be used to develop a personalized treatment plan that includes both conventional and complementary therapies.
For example, an AI based emergency system was integrated in elderly patients homes in Switzerland and used as a 24/7 emergency response operator. This device was placed in multiple rooms of a patient’s home and created daily patterns of an individual by establishing a daily activity pattern using imaging detection of the human moving from room to room. This device was able to distinguish between everyday activities, pets, and falls without a camera or microphone. If a fall is detected or a change is observed in the daily pattern, the detector contacts in a connected app receive an alert. If a contact doesn’t respond to the alert, a call goes out to a 24/7 emergency response operator.
Enhancing Patient Outcomes:
Finally, machine learning can be used to enhance patient outcomes in integrative healthcare. By analyzing data on patient outcomes, machine learning algorithms can identify factors that contribute to successful outcomes or factors that contribute to increased risk for complications and use this information to improve patient care.
For example, machine learning can be used to identify patients who are at high risk of developing complications or experiencing adverse events. By analyzing data on patient outcomes, including hospital readmissions, adverse drug events, and other indicators of poor outcomes, machine learning algorithms can predict which patients are at high risk and develop personalized prevention plans to reduce that risk.
Machine learning can also be used to monitor patient progress and adjust treatment plans as needed. By analyzing data on patient outcomes over time, including changes in symptoms, lab results, and other indicators of disease progression, machine learning algorithms can predict which treatments are most effective for each individual and adjust treatment plans accordingly. When machine learning algorithms are applied to continuous data, such as vitals signs or ECG waveforms, a small change which would normally be dismissed could be an indication of increased risk of complication or arrhythmia. This can lead to better patient outcomes and a higher quality of life.
Finally, machine learning can be used to improve patient engagement and self-management. By analyzing data on patient behavior, including exercise habits, nutrition, and medication adherence, machine learning algorithms can predict which patients are at risk of developing complications and develop personalized interventions to improve self-management. This can include reminders to take medication, exercise, or follow a healthy diet, as well as coaching and support to help patients stay on track.
Challenges and Limitations:
While machine learning has the potential to revolutionize integrative healthcare, there are several challenges and limitations that must be addressed. One of the main challenges is data quality and accessibility. Machine learning algorithms require large amounts of high-quality data to be effective, but much of the data in healthcare is fragmented, incomplete, or difficult to access. Additionally, there are concerns about privacy and security, as patient data is highly sensitive and must be protected from unauthorized access or disclosure.
Another challenge is the complexity of healthcare. Healthcare is a highly complex and dynamic system that involves many different stakeholders, including patients, healthcare providers, insurers, and policymakers. Machine learning algorithms must be able to navigate this complexity and account for the many different factors that can influence patient outcomes.
Finally, there are concerns about the accuracy and reliability of machine learning algorithms. Machine learning algorithms are only as good as the data they are trained on, and biases or errors in the data can lead to inaccurate or unreliable predictions. Additionally, machine learning algorithms are often viewed as a “black box” that is difficult to understand or interpret, which can make it difficult for healthcare providers and patients to trust the recommendations they provide.
Conclusion:
Integrative healthcare is a patient-centered approach that recognizes the importance of personalized care, effective diagnosis and treatment, and patient outcomes. Machine learning has the potential to revolutionize integrative healthcare by providing healthcare providers with the tools they need to analyze large amounts of data, identify patterns and relationships that are not immediately apparent, and develop personalized treatment plans that maximize the chances of success and minimize side effects.
While there are challenges and limitations to the use of machine learning in integrative healthcare, these can be addressed through a combination of technical solutions, policy changes, and stakeholder engagement. By leveraging the power of machine learning to improve patient outcomes, we can create a more effective, efficient, and sustainable healthcare system that meets the needs of all patients.
References:
Sidey-Gibbons JAM, Sidey-Gibbons CJ. Machine learning in medicine: a practical introduction. BMC Med Res Methodol. 2019;19(1):64. Published 2019 Mar 19. doi:10.1186/s12874-019-0681-4
Choi RY, Coyner AS, Kalpathy-Cramer J, Chiang MF, Campbell JP. Introduction to Machine Learning, Neural Networks, and Deep Learning. Transl Vis Sci Technol. 2020;9(2):14. Published 2020 Feb 27. doi:10.1167/tvst.9.2.14
Silva GFS, Fagundes TP, Teixeira BC, Chiavegatto Filho ADP. Machine Learning for Hypertension Prediction: a Systematic Review. Curr Hypertens Rep. 2022;24(11):523-533. doi:10.1007/s11906-022-01212-6
Ananth S. Applying integrative healthcare. Explore (NY). 2009;5(2):119-120. doi:10.1016/j.explore.2008.12.011
Kreitzer MJ, Kligler B, Meeker WC. Health professions education and integrative healthcare. Explore (NY). 2009;5(4):212-227. doi:10.1016/j.explore.2009.05.012
Ngiam KY, Khor IW. Big data and machine learning algorithms for health-care delivery [published correction appears in Lancet Oncol. 2019 Jun;20(6):293]. Lancet Oncol. 2019;20(5):e262-e273. doi:10.1016/S1470-2045(19)30149-4