Problems of Bringing Machine Learning to The Bedside in Healthcare
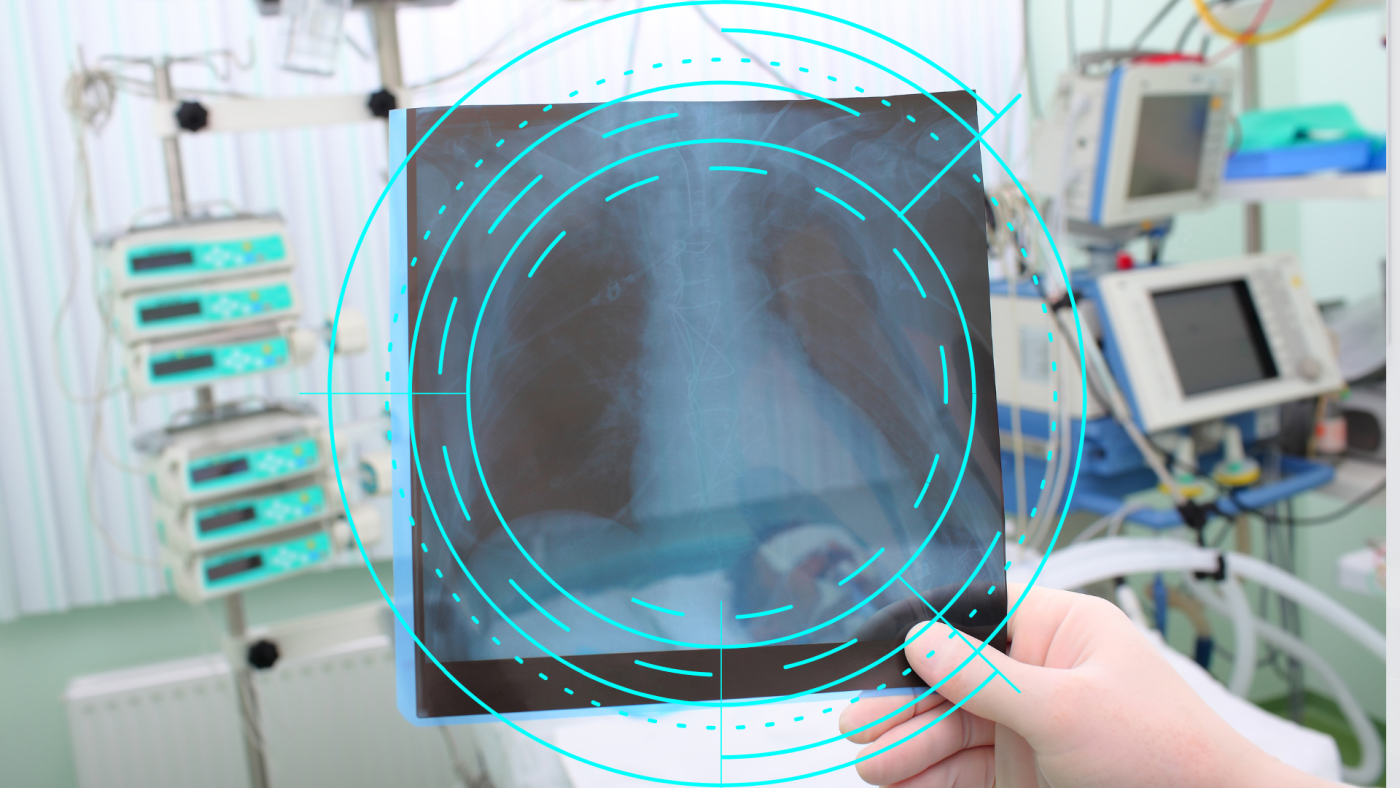
Authors: Amina Khalpey, PhD, Ujjawal Kumar, BA, Ezekiel Mendoza, BS, Zain Khalpey, MD, PhD, FACS
With the development and advances in AI technology, its potential utility in healthcare at the bedside is apparent. However, there are multiple problems with its implementation at the bedside.
The Challenge of Integrating AI With Existing Healthcare Infrastructure
One significant challenge is the integration of AI systems into pre-existing healthcare systems and workflows, which are well-established, and in which healthcare professionals are trained for and comfortable using. This integration will require a significant amount of time, resources, and effort involving both technical specialists and input from stakeholders who will use these systems on a day-to-day basis. Integration may also be complicated by the diversity of healthcare systems and data formats. Furthermore, the compatibility and interoperability of different AI systems is often a challenge, as these systems may not be able to communicate with each other effectively. This can lead to errors and delays in patient care, as well as inefficiencies in the healthcare system at large.
Shortage of AI-trained Healthcare Professionals
Another challenge is the shortage of trained professionals with the skills and knowledge required to implement and use AI in healthcare. There is currently a profound need for healthcare professionals who are trained in and competent in use of AI, as well as those who are aware of the ethical and legal implications of its use. A recent study showed that only 30% of healthcare students and 50% of academic faculty responded that they were aware of AI topics in medicine, with the majority of their subject knowledge coming from the mainstream media. This shortage may lead to slow adoption of AI in healthcare, as well as the use of AI by untrained or unqualified individuals, which can have serious consequences for patients. There is, however, promise; the same study showed that there was almost unanimous interest across students and faculty in wanting to learn more about the field, with interest in healthcare-specific training in AI.
Heterogenous AI Practice Complicates Implementation
A lack of standardization is another challenge facing AI in healthcare. There are currently no established and accepted standards or guidelines for the development, implementation, and use of AI in healthcare. This can result in the creation and simultaneous existence of multiple AI systems that perform the same tasks in different ways, leading to confusion, errors, and inefficiencies. Furthermore, the lack of standardization can make it difficult for healthcare providers to compare and evaluate different AI systems, and thus create difficulty in informed decision making about which systems to use.
Privacy And Data Security Must Remain of Utmost Importance
Another worry with the use of AI in healthcare that must not be overlooked is that of privacy and security. Personal health information is, by definition, highly sensitive and confidential, and therefore must be protected from unauthorized access, use, and disclosure. This is currently possible with human analysis and use of electronic medical record systems, however the emerging role of AI in medicine threatens this data security. The use of AI in healthcare requires large amounts of data to be collected, stored, and analyzed, which can greatly increase the risk of data breaches as well as other security incidents. Like with other computer systems, AI systems may well be vulnerable to hacking and other forms of cyberattacks, which can compromise the privacy and security of patient information, while data security protocols are not yet developed for this novel technological approach to healthcare.
In conclusion, while AI in healthcare has the potential to greatly improve the quality of patient care, there are multiple challenges and problems that must be addressed before it can be effectively implemented at the bedside, a few of which we have described in this essay. These include accuracy, explainability, bias, cost, integration, workforce training, standardization, and privacy and security. Addressing these issues will require a collaborative effort by healthcare organizations, technology companies, and policymakers, as well as significant investment in research and development. Ultimately, the goal must be to develop AI systems that are safe, effective, and trustworthy, and that can be integrated seamlessly into the existing healthcare system to provide the best possible care for patients.
References:
Koski E, Murphy J. AI in Healthcare. Stud Health Technol Inform. 2021 Dec 15;284:295-299. doi: 10.3233/SHTI210726. PMID: 34920529.
Bohr A, Memarzadeh K. The rise of artificial intelligence in healthcare applications. Artificial Intelligence in Healthcare. 2020:25–60. doi: 10.1016/B978-0-12-818438-7.00002-2. Epub 2020 Jun 26. PMCID: PMC7325854.
Wood EA, Ange BL, Miller DD. Are We Ready to Integrate Artificial Intelligence Literacy into Medical School Curriculum: Students and Faculty Survey. J Med Educ Curric Dev. 2021 Jun 23;8:23821205211024078. doi: 10.1177/23821205211024078. PMID: 34250242; PMCID: PMC8239949.
Ahuja AS. The impact of artificial intelligence in medicine on the future role of the physician. PeerJ. 2019 Oct 4;7:e7702. doi: 10.7717/peerj.7702. PMID: 31592346; PMCID: PMC6779111.
Murdoch B. Privacy and artificial intelligence: challenges for protecting health information in a new era. BMC Med Ethics. 2021 Sep 15;22(1):122. doi: 10.1186/s12910-021-00687-3. PMID: 34525993; PMCID: PMC8442400.