Proposed Machine Learning Strategy to Predict Longevity
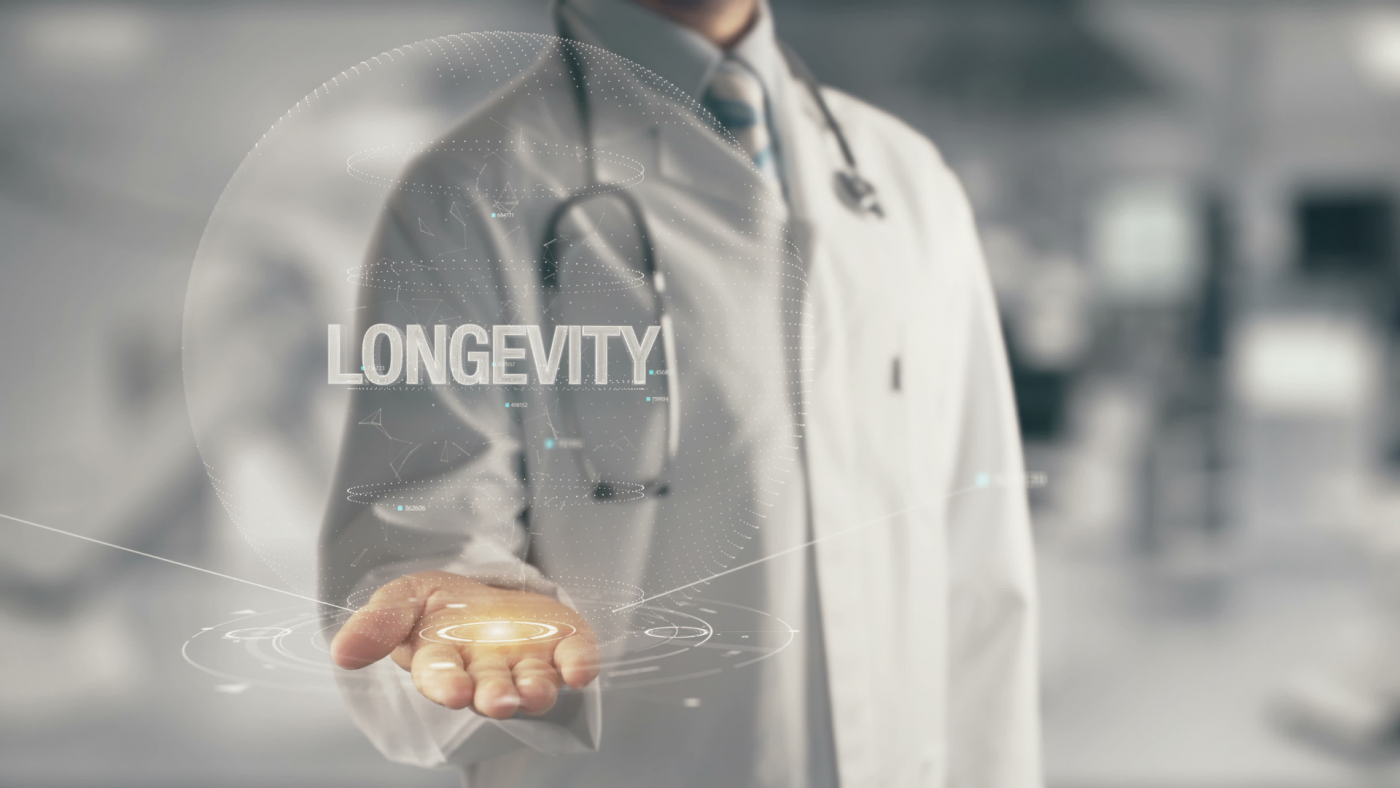
Authors: Amina Khalpey, PhD, Parker Wilson, BS, Brynne Rozell, BS, Zain Khalpey, MD, PhD, FACS,
It is now possible to develop a holistic health assessment and prognostic tool for patients of all demographics by developing personalized machine learning algorithms (MLAs) for longevity. By this we mean that algorithms can be created to analyze a person’s current health and predict incidence and/or progression of disease and even lifespan, if given enough data. Generally, these MLAs would take into account various factors such as lifestyle, genetics, and health history and provide prognostics and suggestions for health and lifestyle improvement. In this blog post, we will use the example of an average middle-aged male to illustrate how longevity can be prognosticated and even extended with the aid of artificial intelligence. When we consider developing such a tool which we have named LIFE (Longevity Informatics Equation), the following strategies are the starting point:
Data collection:
The first step in developing algorithms for longevity is to collect and integrate data from various sources. This data can include the individual’s demographics, health records, family history, personal risk factors, activity level, genetic testing results, and even data from wearable devices, such as smartwatches and fitness trackers. By integrating these different data sources, a comprehensive health profile can be created that provides a well-rounded image of the individual’s health on which projections can be made.
Risk Assessment:
Once the data has been collected, machine learning algorithms (MLAs) would analyze and assess the individual’s risk of developing age-related diseases. MLAs identify patterns and correlations in data that may not be obvious. These algorithms could be integrated with evidence-based high-fidelity risk assessment tools already in use like ASCVD and ADA Diabetes Risk Assessment to better predict morbidity and mortality. Many of these evidence-based medicine (EBM) tools are backed with good, high-powered studies and would lend more credibility to the MLAs assessments.
Personalized Interventions
Based on the results of the risk assessment, personalized interventions can be developed that are tailored to the individual’s specific health profile. These interventions can include dietary changes, exercise routines, medication, and other lifestyle modifications. Resources could even be provided to support these interventions and propel the individual to reach their health goals.
Monitoring and Feedback:
To monitor the individual’s progress, continued data collection would be required including updated health records and evaluation of suggested lifestyle improvements. If data was continually added to the algorithms, they would trend toward increased fidelity and, therefore, reliability. For example, if the individual’s data indicates that a patient’s hypertension is worsening, the algorithm can provide suggestions to start or increase medications. The same algorithm could also provide up-to-date prognostics for worsening hypertension.
Long-term Planning:
Ongoing data collection with MLAs could suggest adjustments to current interventions and make long-term planning and health maintenance more manageable. The data collected could include longitudinal tracking of vital signs, lab work and imaging as regular maintenance of an individual’s medical conditions. Continual data collection for individuals will allow for more and more useful prognostics.
We believe the following list could be useful inputs into algorithms for our proposed LIFE formula.
Evidence-Based Risk Assessment Tools
We have compiled a list of risk-assessment tools that are currently being used in practice which could be incorporated into our algorithms.
Proposed Interventions:
We have also compiled a list of proposed interventions which could be strategically employed to extend longetivity.
In conclusion, with this general outline, algorithms could be devised to provide a well-rounded assessment of the current health and future health outcomes for an average middle-age male, in essence projecting his longevity. This would help to identify the risk and/or severity of age-related diseases and develop personalized interventions to optimize health and extend lifespan. The LIFE Formula would serve as a useful tool for both providers and patients as a way to improve health in a holistic manner. If this tool were eventually made widely available, it would give the public better agency in improving their lives.