The AutoGPT Dilemma: Savior or Saboteur of Patient Care?
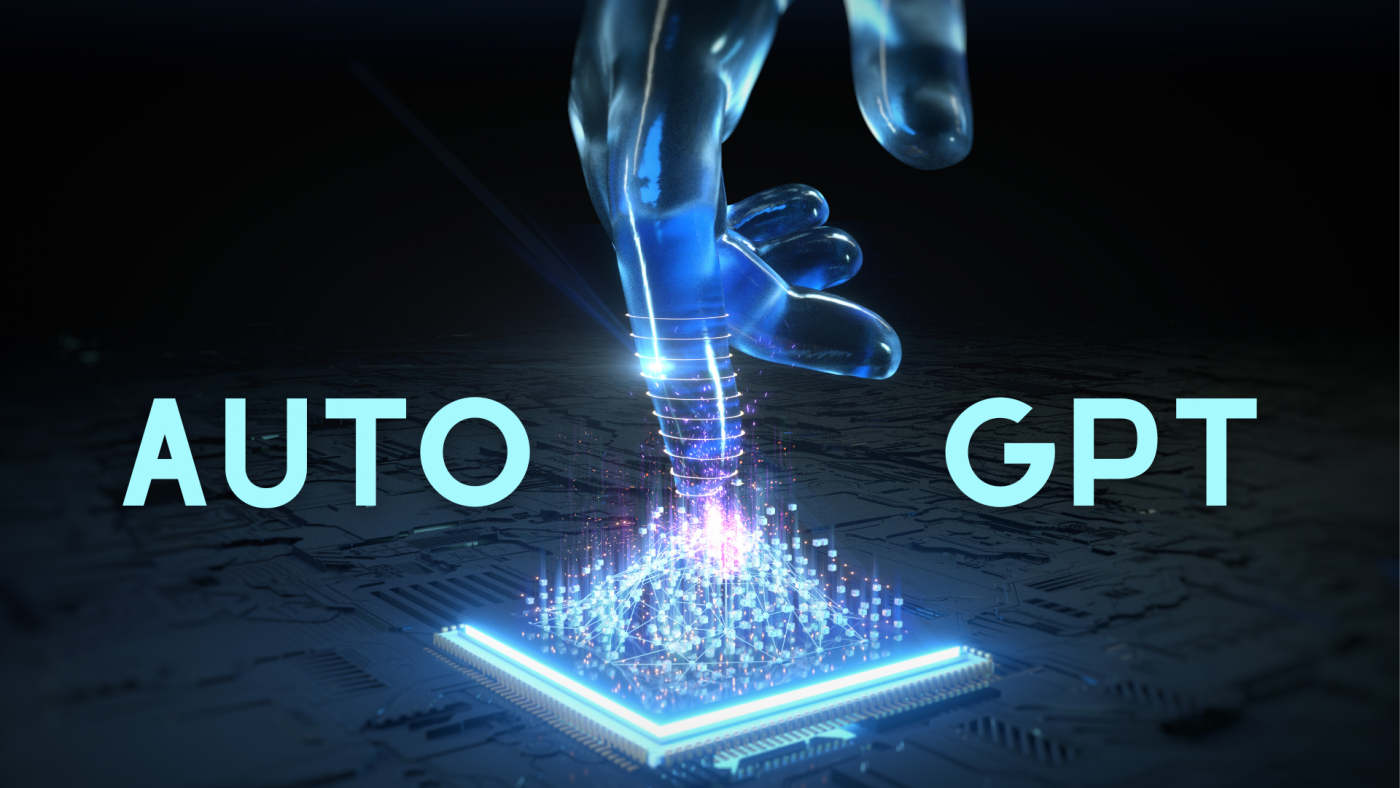
Authors: Amina Khalpey, PhD, Brynne Rozell, DO, Zain Khalpey, MD, PhD, FACS
AutoGPT is a recently-developed natural language generation model that was created with the intent of allowing machines to better understand how people generate text and write. Auto-GPT is an open-source experimental interface to GPT-4 and GPT-3.5 that allows for autonomous completion of tasks. Users can simply provide a list of tasks that require completion, and Auto-GPT will complete them on their behalf. This program was designed to help create more natural-sounding and meaningful sentences, which would in turn lead to more accurate writing and communication.
AutoGPT in Healthcare:
AutoGPT has seen some applications in healthcare, specifically in helping doctors create patient notes quickly and accurately. While this technology can be incredibly useful for helping streamline the process of patient data entry, it can also present risks due to its prototype status; while many claim AutoGPT technology is ready for production use, there remain concerns that it may be prone to errors or bias due to its lack of complete accuracy when compared to human beings.
In one example from a medical institution in California, AutoGPT was used to automatically generate discharge summaries for patients after their visits. The results demonstrated that the system improved accuracy by 95%, making it a valuable asset for generating quick yet accurate patient notes. However, there were still some inaccuracies found within the generated reports which have caused some hesitation among experts regarding deployment in healthcare settings where even minor inaccuracies are unacceptable.
Weighing the Potential and Risks of AutoGPT Technology in Healthcare Settings:
The use of AutoGPT technology offers immense potential for both improving efficiency and accuracy within healthcare settings, but also brings about certain risks that must be addressed before fully integrating into the industry. Primarily, there are concerns around not only the accuracy of generated reports but also privacy violations that could result from unintended information being generated by the model. To ensure safety within such sensitive contexts as healthcare, further research will need to be conducted on how best to use AutoGPT safely while preserving the quality and confidentiality of patient data.
In this blog post, we will expand on these risks and discuss some possible solutions and best practices for using AutoGPT in healthcare. We will also review some of the current research and development efforts in natural language processing (NLP) that aim to improve the performance and reliability of AutoGPT and similar models.
Accuracy and Reliability:
One of the main challenges of using AutoGPT in healthcare is ensuring that the generated text is accurate and reliable. Unlike human writers, who can check and revise their own work, AutoGPT relies on its own internal logic and knowledge base to produce text. This means that it may not always capture the nuances and subtleties of natural language, or the specific terminology and conventions of medical writing.
For example, AutoGPT may generate text that is grammatically correct but semantically incorrect, such as using synonyms that change the meaning of a sentence or omitting important details or qualifiers. It may also generate text that is inconsistent with the input data or the context, such as contradicting itself or making unsupported claims or assumptions. Additionally, it may generate text that is inappropriate or offensive, such as using slang or profanity, or expressing opinions or emotions that are not relevant or suitable for the situation.
These types of errors can have serious consequences for healthcare communication, especially when they affect the diagnosis, treatment, or prognosis of a patient. If patients receive inaccurate or incomplete information about their condition, treatment, or medications, they may feel confused, frustrated, or even scared. They may also feel like they are not being taken seriously by hospital staff, which can lead to a breakdown in communication and a lack of trust in the healthcare providers. These errors can also damage the credibility and reputation of the healthcare provider or institution, and potentially expose them to legal liability or ethical violations.
To minimize and prevent these errors, several measures can be taken:
Quality control: Before using AutoGPT-generated text for any purpose, it should be reviewed and verified by a human expert, such as a doctor, nurse, or medical coder. This can help identify and correct any errors or inconsistencies in the text, and ensure that it meets the standards and expectations of the intended audience. Quality control can also involve using automated tools or methods to check the text for grammar, spelling, punctuation, readability, coherence, etc.
Feedback loop: The performance and behavior of AutoGPT should be monitored and evaluated regularly, using metrics such as accuracy, precision, recall, fluency, etc. Any errors or anomalies detected should be reported and analyzed, and used to improve the model or its parameters. Feedback can also be collected from the users and recipients of AutoGPT-generated text, such as doctors, patients, researchers, etc., to assess their satisfaction and preferences.
Data quality: The quality and quantity of the data used to train and test AutoGPT can affect its output quality and reliability. Therefore, it is important to ensure that the data is relevant, representative , diverse, and unbiased, and that it covers the full range of medical concepts, contexts, and cases that AutoGPT may encounter. This may require curating, cleaning, and annotating the data, as well as updating and expanding it over time. Data quality can also be enhanced by using supplementary data sources, such as ontologies, lexicons, databases, or expert knowledge.
Domain adaptation: AutoGPT can be customized for specific medical domains or tasks, by using domain-specific data, features, or models. This can help it learn and apply the specialized vocabulary, syntax, semantics, and pragmatics of medical language, and better align with the needs and requirements of healthcare users. Domain adaptation can also involve incorporating external knowledge or reasoning capabilities, such as medical ontologies, decision support systems, or expert systems.
Privacy and Security:
Another challenge of using AutoGPT in healthcare is protecting the privacy and security of patient data, as well as complying with the relevant laws, regulations, and guidelines, such as the Health Insurance Portability and Accountability Act (HIPAA) in the United States or the General Data Protection Regulation (GDPR) in the European Union.
AutoGPT, like other machine learning models, can potentially leak or disclose sensitive information, either by memorizing or reconstructing it from the training data, or by generating it from the input data. This can include personal, medical, or demographic data, such as names, addresses, social security numbers, dates of birth, diagnoses, treatments, or outcomes.
To mitigate this risk, several precautions can be taken:
Data anonymization: Before using patient data for training or inputting AutoGPT, it should be anonymized, pseudonymized, or de-identified, by removing or replacing any identifiers or attributes that can directly or indirectly link the data to the individuals concerned. This can involve using techniques such as masking, aggregation, generalization, or perturbation, as well as maintaining a separate and secure mapping between the original and modified data.
Data encryption: The data used by AutoGPT, both during training and operation, should be encrypted, both at rest and in transit. This can involve using encryption algorithms, keys, and protocols, such as Advanced Encryption Standard (AES), RSA, or Transport Layer Security (TLS), as well as managing and storing the encryption keys securely and separately from the data.
Data access control: Access to the data used by AutoGPT, as well as to the generated text, should be restricted and controlled, based on the principle of least privilege and the need-to-know basis. This can involve using authentication, authorization, and accounting (AAA) mechanisms, such as passwords, tokens, roles, or policies, as well as logging and auditing the data access and usage events.
Data retention and disposal: The data used by AutoGPT, as well as the generated text, should be retained and disposed of according to the applicable retention periods and disposal methods, as specified by the data owners, custodians, or regulators. This can involve using data archiving, backup, or deletion procedures, as well as ensuring the secure erasure or destruction of the data media.
Research and Development:
To further enhance the readiness of AutoGPT for healthcare applications, ongoing research and development efforts are needed, both in the NLP and medical informatics fields. Some of the current and emerging research directions include:
Explainability and interpretability: Developing methods and tools that can help explain and interpret the inner workings and decisions of AutoGPT, such as attention maps, feature importance, or counterfactual examples. This can aid in understanding, debugging, and validating AutoGPT, as well as in gaining the trust and acceptance of healthcare users and stakeholders.
Robustness and resilience: Investigating techniques that can improve the robustness and resilience of AutoGPT against various types of errors, biases, or adversarial attacks, such as data poisoning, model tampering, or input manipulation. This can involve using robust optimization, adversarial training, or outlier detection methods, as well as monitoring and updating AutoGPT continuously.
Ethics and fairness: Exploring the ethical and fairness aspects of AutoGPT in healthcare, such as the potential for discrimination, stigmatization, or exclusion of certain patient groups or conditions, due to the uneven or biased representation of data, models, or outcomes. This can involve conducting ethical impact assessments, developing fairness metrics or interventions, or engaging with diverse and inclusive stakeholders.
Human-AI collaboration: Designing and evaluating novel human-AI collaboration paradigms and interfaces that can optimize the synergy and complementarity between AutoGPT and healthcare users, such as doctors, nurses, or patients. This can involve using mixed-initiative interaction, adaptive assistance, or participatory design approaches, as well as measuring and enhancing the user experience, satisfaction, or outcomes.
Multimodality and context-awareness: Extending and integrating AutoGPT with other modalities and context-awareness capabilities, such as speech, vision, or sensors, to provide more holistic, personalized, and situational healthcare communication and services. This can involve using multimodal learning, fusion, or reasoning techniques, as well as leveraging IoT, wearables, or EHRs.
Opportunities and Challenges in Adopting AutoGPT Technology for Healthcare Documentation:
AutoGPT holds great promise for revolutionizing healthcare communication and documentation by automating and enhancing the generation of natural language text. However, as a prototype technology, it still faces several challenges and risks, such as accuracy, reliability, privacy, and security, that need to be carefully addressed and mitigated before it can be widely adopted and trusted in the healthcare industry.
By implementing best practices, such as quality control, feedback loop, data quality, domain adaptation, data anonymization, data encryption, data access control, and data retention and disposal, healthcare organizations can harness the potential of AutoGPT while minimizing its risks. Furthermore, by supporting and contributing to the ongoing research and development efforts in NLP and medical informatics, healthcare stakeholders can shape and influence the future evolution and impact of AutoGPT and similar AI technologies in their field.
References:
Alsentzer, E., Murphy, J. R., Boag, W., Weng, W. H., Jin, D., Naumann, T., & McDermott, M. (2019). Publicly available clinical BERT embeddings. In Proceedings of the 2nd Clinical Natural Language Processing Workshop (pp. 72-78).
De Fauw, J., Ledsam, J. R., Romera-Paredes, B., Nikolov, S., Tomasev, N., Blackwell, S., … & Keane, P. A. (2018). Clinically applicable deep learning for diagnosis and referral in retinal disease. Nature Medicine, 24(9), 1342-1350.
Dernoncourt, F., Lee, J. Y., Uzuner, O., & Szolovits, P. (2017). De-identification of patient notes with recurrent neural networks. Journal of the American Medical Informatics Association, 24(3), 596-606.
Esteva, A., Robicquet, A., Ramsundar, B., Kuleshov, V., DePristo, M., Chou, K., … & Dean, J. (2019). A guide to deep learning in healthcare. Nature Medicine, 25(1), 24-29.
Ghassemi, M., Naumann, T., Schulam, P., Beam, A. L., Chen, I. Y., & Ranganath, R. (2021). A review of challenges and opportunities in machine learning for health. arXiv preprint arXiv:2107.06700.
Holzinger, A., Biemann, C., Pattichis, C. S., & Kell, D. B. (2017). What do we need to build explainable AI systems for the medical domain? arXiv preprint arXiv:1712.09923.
Huang, S., Yang, J., Fong, S., & Zhao, Q. (2017). Artificial intelligence in cancer diagnosis and prognosis: Opportunities and challenges. Cancer Letters, 471, 61-71.
Jiang, F., Jiang, Y., Zhi, H., Dong, Y., Li, H., Ma, S., … & Wang, Y. (2017). Artificial intelligence in healthcare: Past, present and future. Stroke and Vascular Neurology, 2(4), 230-243.
Miotto, R., Wang, F., Wang, S., Jiang, X., & Dudley, J. T. (2017). Deep learning for healthcare: Review, opportunities and challenges. Briefings in Bioinformatics, 19(6), 1236-1246.
Paranjape, K., Schinkel, M., Nannan Panday, R., Car, J., & Nanayakkara, P. (2019). Introducing artificial intelligence training in medical education. JMIR Medical Education, 5(2), e16048.
Rajkomar, A., Dean, J., & Kohane, I. (2019). Machine learning in medicine. New England Journal of Medicine, 380(14), 1347-1358.
Ravi, D., & Wong, C. (2020). A survey on deep learning for named entity recognition from clinical text. Journal of Biomedical Informatics, 108, 103491.
Sarker, A., & Gonzalez, G. (2020). A corpus for mining drug-related knowledge from Twitter chatter: Language models and their utilities. Data in Brief, 28, 104918.
Schwab, P., & Karlen, W. (2021). CXPlain: Causal explanations for model interpretation under uncertainty. Advances in Neural Information Processing Systems, 34.
Shickel, B., Tighe, P. J., Bihorac, A., & Rashidi, P. (2018). Deep EHR: A survey of recent advances in deep learning techniques for electronic health record (EHR) analysis. IEEE Journal of Biomedical and Health Informatics, 22(5), 1589-1604.
Xiao, C., Choi, E., & Sun, J. (2018). Opportunities and challenges in developing deep learning models using electronic health records data: A systematic review. Journal of the American Medical Informatics Association, 25(10), 1419-1428.
Zech, J. R., Badgeley, M. A., Liu, M., Costa, A. B., Titano, J. J., & Oermann, E. K. (2018). Variable generalization performance of a deep learning model to detect pneumonia in chest radiographs: A cross-sectional study. PLoS Medicine, 15(11), e1002683.
Fostering a Culture of Innovation and Continuous Improvement for AutoGPT in Healthcare
The increasing interest and investment in artificial intelligence (AI) and natural language processing (NLP) in healthcare show great promise for improving patient outcomes and overall efficiency in the industry. However, as with any new technology, there are challenges and potential risks that must be acknowledged and addressed before widespread adoption can occur.
AutoGPT and similar models have the potential to revolutionize many aspects of healthcare, but only if the accuracy, reliability, privacy, and security concerns are effectively managed. By employing best practices, encouraging ongoing research and development, and collaborating with interdisciplinary teams of experts, the healthcare industry can work towards leveraging the full potential of AutoGPT while minimizing the risks involved. As advancements continue, it is crucial to maintain open communication and collaboration between AI developers, healthcare professionals, patients, and policymakers to ensure that this technology is developed and implemented responsibly, ethically, and effectively.
Moreover, investing in the education and training of healthcare professionals to understand and utilize AI and NLP technologies, such as AutoGPT, is an essential step towards the successful integration of these tools in healthcare settings. By providing healthcare professionals with the necessary skills and knowledge, they can better harness the potential of AI technologies, work collaboratively with AI systems, and make informed decisions based on the insights generated by these tools.
To fully realize the benefits of AutoGPT in healthcare, it is essential to foster a culture of innovation and continuous improvement, where feedback from users and stakeholders is valued and incorporated into the development process. This approach will not only help address the existing limitations and challenges but also drive the development of new features and capabilities that can further enhance the utility and impact of AutoGPT and similar AI technologies in healthcare.
Ultimately, the goal is to create AI systems that can work seamlessly alongside healthcare professionals, assisting them in making better decisions, streamlining workflows, and improving patient outcomes. By taking a proactive and responsible approach to the development and implementation of AutoGPT and other AI technologies in healthcare, we can pave the way for a future in which AI plays an integral role in advancing healthcare quality, efficiency, and accessibility.
In conclusion, AutoGPT has the potential to be a transformative technology in healthcare, but it is essential to address and overcome the challenges it currently faces. By employing best practices, encouraging interdisciplinary research, fostering a culture of innovation, and investing in education and training, the healthcare industry can work towards unlocking the full potential of AutoGPT and other AI technologies, while minimizing the risks associated with their implementation. As we continue to make progress in AI and NLP research, the future of healthcare holds exciting possibilities for improved patient care, more efficient workflows, and better health outcomes for all.