Predict and Prevent: Artificial Intelligence in Medicine
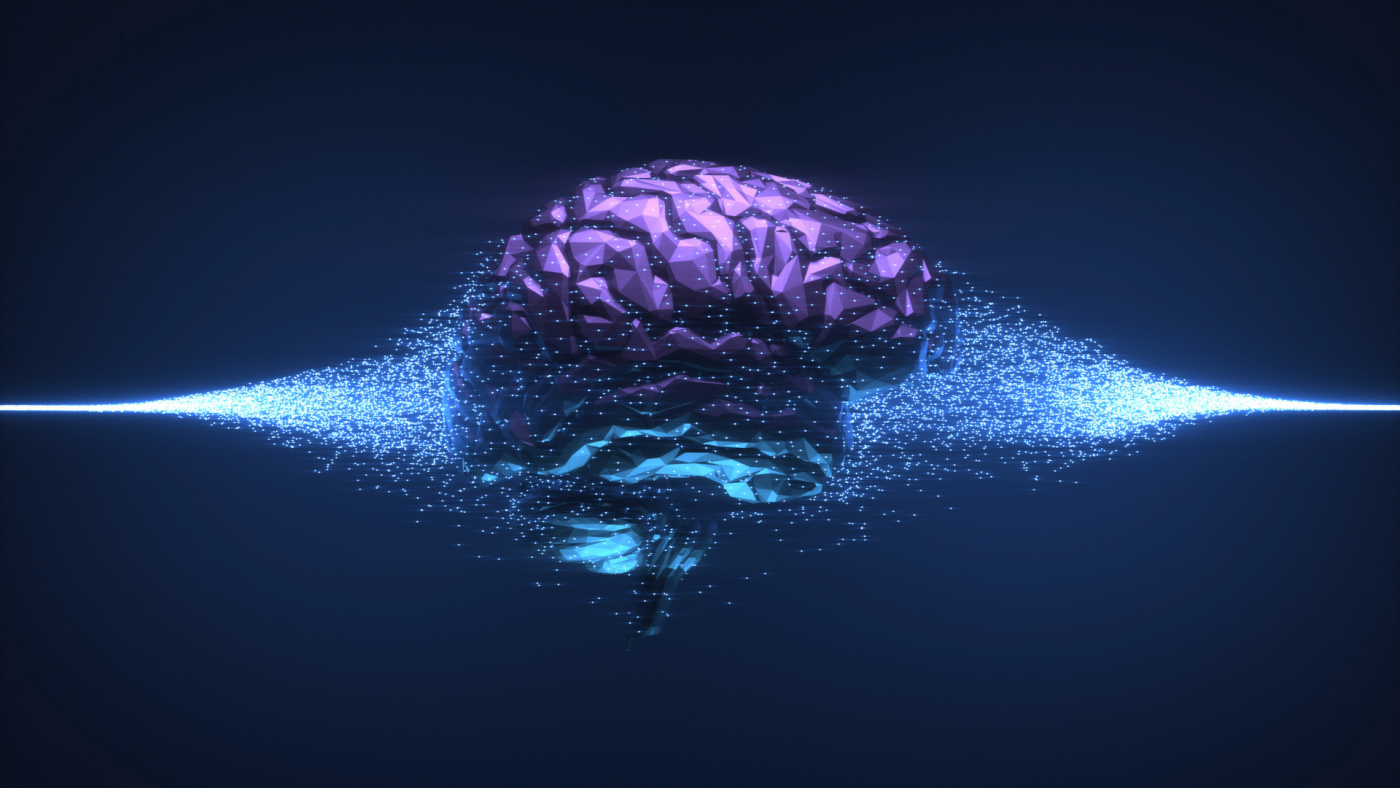
Authors: Amina Khalpey, PhD, Parker Wilson, DO, Zain Khalpey, MD, PhD, FACS
Disease prevention is the pinnacle of modern medicine. We as physicians want to predict and prevent any possible disease process before it creates detriment for our patients yet there are limits to our ability to do so. Artificial Intelligence (AI), however, is beginning to challenge this frontier of medicine. AI technologies like machine learning (ML) have shown significant potential to identify patterns and trends in patient data to anticipate disease, just as clinicians do but more quickly and accurately. With the use of AI, predictive and preventative healthcare could grow and revolutionize modern medicine with significant advances in patient care and outcomes. In this essay, we will explore the potential of AI in predictive and preventative healthcare, its current limitations, and the ethical concerns surrounding its use.
Utility:
Specifically, AI technologies using predictive analytics, which involves the analysis of historical data to make predictions about future outcomes, could help healthcare providers to identify patients who are at risk of developing a particular condition. By identifying at-risk patients, healthcare providers can take proactive measures to prevent the onset of the disease, leading to better health outcomes.
Although physicians and scientists have long analyzed data retrospectively to create predictive algorithms, such as ASCVD and STS equation, the process of their creation is arduous and requires massive amounts of high-quality data. AI and Machine learning will invigorate and speed-up this process. Machine learning (ML) algorithms can be developed to analyze large data sets and report patterns and trends that would take much longer for humans to calculate. By analyzing electronic health records, genomic data, and other sources of patient data, AI can identify risk factors and predict the likelihood of certain conditions. Posts on our blog detail the current applications of AI predict cardiac pathologies like arrhythmia and heart failure. Heart complication prediction and Anticipating Cardiovascular Disease are two fascinating posts about these applications.
AI can also assist healthcare providers in the diagnosis of conditions. By analyzing images and other medical data, AI algorithms can detect patterns quickly and accurately that may be difficult for humans to recognize. For example, AI can assist in the diagnosis of skin cancer by analyzing images of skin lesions. There are studies that have shown ML algorithms useful in Differentiating Malignant and Benign Lung Nodules. AI can also assist in the diagnosis of heart disease and atrial fibrillation by Analyzing EKG Data.
By using precise predictive models like the ones linked above, clinicians can anticipate disease and treat patients well before they develop signs and symptoms. This will undoubtedly improve patient care and outcomes, but there are limitations to this technology as it currently stands.
Limitations:
Despite its potential, AI in predictive and preventative healthcare has its limitations. One of the most significant limitations is the lack of access to high-quality patient data. While electronic health records and other sources of patient data are becoming more widely available, there are still significant gaps in the data that is available. Without access to high-quality data, AI algorithms will not be able to identify patterns and trends accurately.
Another limitation of AI in predictive and preventative healthcare is the potential for bias in the algorithms. ML algorithms are only as free from bias as the data that they are trained on. If the data used to train the algorithms is biased, the algorithms will also be biased. This could lead to incorrect predictions and treatment plans. AI algorithms also have limitations in their ability to handle complex data. While AI can analyze vast amounts of data, it may not be able to handle complex data sets that require a high degree of interpretation. For example, AI algorithms may struggle to analyze medical imaging data that requires a high degree of expertise to interpret.
Finally, the use of AI raises ethical concerns. One of the most significant concerns is the potential for privacy violations. The use of electronic health records and other sources of patient data raises questions about who has access to the data and how it is used. There is also a concern about the potential for discrimination based on the data that is analyzed.
Ethical Concerns:
The ethical implications for broad use of AI in healthcare can be broken down into privacy, concerns and discrimination.
Data Privacy:
Whenever patient data is accessed, there is concern for privacy. Personal Health Information (PHI) must be protected to prevent access by anyone but the patient and those involved in patient care. The use of electronic health records and other sources of patient data to train AI algorithms, therefore, raises concerns about data privacy. Healthcare providers and researchers must ensure that patient data is collected and used in a manner that is consistent with ethical standards and legal regulations. Patients have the right to know how their data is being used and to provide informed consent for its use.
Even when the data is collected with consent by patients, the collection, analysis and storage of patient data must remain secure. Healthcare providers must ensure that patient data is protected from unauthorized access or disclosure. This includes implementing appropriate security measures and ensuring that all personnel who have access to patient data are trained in data security protocols. There must also be multiple safeguards in place to constantly monitor data security.
Consent:
Consent is paramount in medicine. So should it also be in training of medical AI. Patients must be informed of how their data will be used and have the right to refuse its use. Consent must be obtained in a manner that is informed and voluntary, and patients must be informed of their right to withdraw their consent at any time.
Potential Discrimination:
Bias and discrimination have hindered high-quality research efforts for decades. And it is also an inherent evil that must also be mitigated when training artificial intelligence. AI algorithms are only as unbiased as the data that they are trained on. If the data used to train the algorithms is biased, the algorithms will also be biased. This could lead to incorrect predictions and treatment plans that are based on factors such as race, ethnicity, or socioeconomic status.
To avoid discrimination, healthcare providers and researchers must ensure that patient data is collected and analyzed in a manner that is unbiased and that takes into account factors such as social determinants of health. AI algorithms must be trained on data that is representative of the population that they are intended to serve, and must be designed to avoid perpetuating biases that are present in the data.
Conclusion:
AI technologies have shown significant potential to identify patterns and trends in patient data to anticipate disease, just as clinicians do but more quickly and accurately. With the use of AI, predictive and preventative healthcare could grow and revolutionize modern medicine with significant advances in patient care and outcomes. However, its use raises significant ethical concerns, particularly in relation to data privacy, consent, and potential discrimination. Healthcare providers and researchers must ensure that patient data is collected and analyzed in a manner that is consistent with ethical standards and legal regulations, and that AI algorithms are designed to avoid perpetuating biases that are present in the data. With appropriate safeguards in place, the use of AI in predictive and preventative healthcare could lead to significant advances in patient care and outcomes.
References:
1. Goff DC Jr, et. al. 2013 ACC/AHA Guideline on the Assessment of Cardiovascular Risk A Report of the American College of Cardiology/American Heart Association Task Force on Practice Guidelines. Circulation. 2014 Jun 24;129(25 Suppl 2):S49-73
2. Shahian DM, Jacobs JP, Badhwar V, Kurlansky PA, Furnary AP, Cleveland JC, Lobdell KW, Vassileva C, Wyler von Ballmoos MC, Thourani VH, Rankin JS, Edgerton JR, D’Agostino RS et al. The Society of Thoracic Surgeons 2018 Adult Cardiac Surgery Risk Models: Part 1—Background, Design Considerations, and Model Development. The Annals of Thoracic Surgery. 2018.
3. Chen M, Decary M. Artificial intelligence in healthcare: An essential guide for health leaders. Healthc Manage Forum. 2020;33(1):10-18.